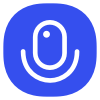
Sign up to save your podcasts
Or
Seventy3: 用NotebookLM将论文生成播客,让大家跟着AI一起进步。
今天的主题是:Inference-Time Scaling for Diffusion Models beyond Scaling Denoising StepsSummary
This research explores enhancing diffusion models by scaling inference-time computation beyond simply increasing denoising steps. The authors propose a search framework that identifies better noises for the diffusion sampling process. This framework considers verifiers for feedback and algorithms to find noise candidates. Experiments on image generation show that increasing inference-time compute through this search framework improves sample quality. The study also analyzes the alignment between verifiers and generation tasks, revealing inherent biases. Ultimately, findings demonstrate substantial improvements in sample generation by diffusion models with increased computing power and a carefully chosen search setup.
这项研究探讨了通过扩大推理时计算量来提升扩散模型的表现,而不仅仅是增加去噪步骤。作者提出了一个搜索框架,用于识别更适合扩散采样过程的噪声。该框架考虑了反馈验证器和算法,用于寻找噪声候选项。图像生成实验表明,通过这一搜索框架增加推理时计算量能够提升样本质量。研究还分析了验证器与生成任务之间的对齐情况,揭示了固有的偏差。最终,研究结果表明,通过增加计算能力和精心选择搜索设置,扩散模型在样本生成方面实现了显著的提升。
原文链接:https://arxiv.org/abs/2501.09732
Seventy3: 用NotebookLM将论文生成播客,让大家跟着AI一起进步。
今天的主题是:Inference-Time Scaling for Diffusion Models beyond Scaling Denoising StepsSummary
This research explores enhancing diffusion models by scaling inference-time computation beyond simply increasing denoising steps. The authors propose a search framework that identifies better noises for the diffusion sampling process. This framework considers verifiers for feedback and algorithms to find noise candidates. Experiments on image generation show that increasing inference-time compute through this search framework improves sample quality. The study also analyzes the alignment between verifiers and generation tasks, revealing inherent biases. Ultimately, findings demonstrate substantial improvements in sample generation by diffusion models with increased computing power and a carefully chosen search setup.
这项研究探讨了通过扩大推理时计算量来提升扩散模型的表现,而不仅仅是增加去噪步骤。作者提出了一个搜索框架,用于识别更适合扩散采样过程的噪声。该框架考虑了反馈验证器和算法,用于寻找噪声候选项。图像生成实验表明,通过这一搜索框架增加推理时计算量能够提升样本质量。研究还分析了验证器与生成任务之间的对齐情况,揭示了固有的偏差。最终,研究结果表明,通过增加计算能力和精心选择搜索设置,扩散模型在样本生成方面实现了显著的提升。
原文链接:https://arxiv.org/abs/2501.09732