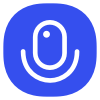
Sign up to save your podcasts
Or
Seventy3: 用NotebookLM将论文生成播客,让大家跟着AI一起进步。
今天的主题是:How to Train Your Energy-Based ModelsSummary
Energy-Based Models (EBMs) offer a flexible approach to probabilistic modeling by specifying probability up to a normalizing constant, enabling the use of versatile architectures. The challenge lies in training these models due to the intractable normalizing constant. This document introduces and compares modern EBM training methods, focusing on Maximum Likelihood with Markov Chain Monte Carlo (MCMC), Score Matching (SM), and Noise Contrastive Estimation (NCE). The document elucidates the theoretical connections among these techniques and briefly explores alternative training methodologies. It also highlights the application of these techniques to score-based generative models. Finally, it discusses minimizing differences or derivatives of KL Divergences, minimizing the Stein discrepancy, and adversarial training.
能量基模型(EBMs)通过指定概率直到归一化常数,提供了一种灵活的概率建模方法,从而能够使用多种架构。训练这些模型的挑战在于归一化常数难以计算。本文介绍并比较了现代EBM训练方法,重点讨论了最大似然估计结合马尔可夫链蒙特卡洛(MCMC)、评分匹配(SM)和噪声对比估计(NCE)。文章阐明了这些技术之间的理论联系,并简要探讨了其他替代训练方法。同时,文章还重点介绍了这些技术在基于评分的生成模型中的应用。最后,本文讨论了最小化KL散度的差异或导数、最小化Stein差异性和对抗性训练的相关内容。
原文链接:https://arxiv.org/abs/2101.03288
Seventy3: 用NotebookLM将论文生成播客,让大家跟着AI一起进步。
今天的主题是:How to Train Your Energy-Based ModelsSummary
Energy-Based Models (EBMs) offer a flexible approach to probabilistic modeling by specifying probability up to a normalizing constant, enabling the use of versatile architectures. The challenge lies in training these models due to the intractable normalizing constant. This document introduces and compares modern EBM training methods, focusing on Maximum Likelihood with Markov Chain Monte Carlo (MCMC), Score Matching (SM), and Noise Contrastive Estimation (NCE). The document elucidates the theoretical connections among these techniques and briefly explores alternative training methodologies. It also highlights the application of these techniques to score-based generative models. Finally, it discusses minimizing differences or derivatives of KL Divergences, minimizing the Stein discrepancy, and adversarial training.
能量基模型(EBMs)通过指定概率直到归一化常数,提供了一种灵活的概率建模方法,从而能够使用多种架构。训练这些模型的挑战在于归一化常数难以计算。本文介绍并比较了现代EBM训练方法,重点讨论了最大似然估计结合马尔可夫链蒙特卡洛(MCMC)、评分匹配(SM)和噪声对比估计(NCE)。文章阐明了这些技术之间的理论联系,并简要探讨了其他替代训练方法。同时,文章还重点介绍了这些技术在基于评分的生成模型中的应用。最后,本文讨论了最小化KL散度的差异或导数、最小化Stein差异性和对抗性训练的相关内容。
原文链接:https://arxiv.org/abs/2101.03288