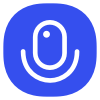
Sign up to save your podcasts
Or
Seventy3: 用NotebookLM将论文生成播客,让大家跟着AI一起进步。
今天的主题是:VideoRAG: Retrieval-Augmented Generation over Video CorpusSummary
VideoRAG is a novel framework that enhances Retrieval-Augmented Generation (RAG) by incorporating video content. Unlike traditional RAG, which primarily uses text, VideoRAG dynamically retrieves relevant videos and integrates both visual and textual information from them to generate more accurate and contextually rich answers. This approach leverages Large Video Language Models (LVLMs) to directly process video content and seamlessly combine it with queries. Experimental results demonstrate VideoRAG's superiority over existing RAG baselines, proving the effectiveness of using videos as a knowledge source. The study also addresses the challenge of missing video subtitles by generating auxiliary text using automatic speech recognition. Finally, the exploration of different modalities and their combinations underscores the importance of both visual and textual features in video-based RAG.
VideoRAG 是一种新型框架,通过引入视频内容增强了检索增强生成(RAG)。与传统的RAG主要依赖文本不同,VideoRAG 动态地检索相关视频,并从中整合视觉和文本信息,以生成更准确、更具上下文丰富性的答案。这一方法利用大型视频语言模型(LVLMs)直接处理视频内容,并将其与查询无缝结合。实验结果表明,VideoRAG 优于现有的RAG基准,证明了使用视频作为知识来源的有效性。该研究还解决了缺失视频字幕的问题,通过自动语音识别生成辅助文本。最后,不同模态及其组合的探索强调了视觉和文本特征在基于视频的RAG中的重要性。
原文链接:https://arxiv.org/abs/2501.05874
Seventy3: 用NotebookLM将论文生成播客,让大家跟着AI一起进步。
今天的主题是:VideoRAG: Retrieval-Augmented Generation over Video CorpusSummary
VideoRAG is a novel framework that enhances Retrieval-Augmented Generation (RAG) by incorporating video content. Unlike traditional RAG, which primarily uses text, VideoRAG dynamically retrieves relevant videos and integrates both visual and textual information from them to generate more accurate and contextually rich answers. This approach leverages Large Video Language Models (LVLMs) to directly process video content and seamlessly combine it with queries. Experimental results demonstrate VideoRAG's superiority over existing RAG baselines, proving the effectiveness of using videos as a knowledge source. The study also addresses the challenge of missing video subtitles by generating auxiliary text using automatic speech recognition. Finally, the exploration of different modalities and their combinations underscores the importance of both visual and textual features in video-based RAG.
VideoRAG 是一种新型框架,通过引入视频内容增强了检索增强生成(RAG)。与传统的RAG主要依赖文本不同,VideoRAG 动态地检索相关视频,并从中整合视觉和文本信息,以生成更准确、更具上下文丰富性的答案。这一方法利用大型视频语言模型(LVLMs)直接处理视频内容,并将其与查询无缝结合。实验结果表明,VideoRAG 优于现有的RAG基准,证明了使用视频作为知识来源的有效性。该研究还解决了缺失视频字幕的问题,通过自动语音识别生成辅助文本。最后,不同模态及其组合的探索强调了视觉和文本特征在基于视频的RAG中的重要性。
原文链接:https://arxiv.org/abs/2501.05874