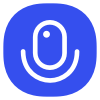
Sign up to save your podcasts
Or
Seventy3: 用NotebookLM将论文生成播客,让大家跟着AI一起进步。
今天的主题是:Embodied-RAG: General Non-parametric Embodied Memory for Retrieval and GenerationSummary
This paper introduces Embodied-RAG, a novel framework designed to equip robots with enhanced memory and reasoning capabilities in complex environments. It tackles challenges in applying Retrieval-Augmented Generation (RAG) to robotics by constructing a hierarchical semantic forest for efficient knowledge storage and retrieval. Embodied-RAG integrates multimodal data and spatial awareness, outperforming existing RAG methods in navigation and explanation tasks. A new dataset, the Embodied-Experiences Dataset, is introduced to facilitate further research in this area. The core innovation lies in the system's ability to build and utilize a hierarchical spatial memory, enabling robots to navigate and communicate more effectively across diverse environments and query types. This work provides a foundation for developing generalist robot agents with language-based non-parametric memories.
本文介绍了Embodied-RAG,这是一种新型框架,旨在赋予机器人在复杂环境中更强的记忆和推理能力。它通过构建一个层次化的语义森林来解决将检索增强生成(RAG)应用于机器人领域的挑战,从而实现高效的知识存储和检索。Embodied-RAG 集成了多模态数据和空间意识,在导航和解释任务中优于现有的RAG方法。文中还引入了一个新的数据集——Embodied-Experiences 数据集,以促进该领域的进一步研究。该系统的核心创新在于其构建和利用层次化空间记忆的能力,使机器人能够更有效地在不同的环境和查询类型中进行导航和交流。这项工作为开发基于语言的非参数记忆的通用机器人智能体奠定了基础。
原文链接:https://arxiv.org/abs/2409.18313
Seventy3: 用NotebookLM将论文生成播客,让大家跟着AI一起进步。
今天的主题是:Embodied-RAG: General Non-parametric Embodied Memory for Retrieval and GenerationSummary
This paper introduces Embodied-RAG, a novel framework designed to equip robots with enhanced memory and reasoning capabilities in complex environments. It tackles challenges in applying Retrieval-Augmented Generation (RAG) to robotics by constructing a hierarchical semantic forest for efficient knowledge storage and retrieval. Embodied-RAG integrates multimodal data and spatial awareness, outperforming existing RAG methods in navigation and explanation tasks. A new dataset, the Embodied-Experiences Dataset, is introduced to facilitate further research in this area. The core innovation lies in the system's ability to build and utilize a hierarchical spatial memory, enabling robots to navigate and communicate more effectively across diverse environments and query types. This work provides a foundation for developing generalist robot agents with language-based non-parametric memories.
本文介绍了Embodied-RAG,这是一种新型框架,旨在赋予机器人在复杂环境中更强的记忆和推理能力。它通过构建一个层次化的语义森林来解决将检索增强生成(RAG)应用于机器人领域的挑战,从而实现高效的知识存储和检索。Embodied-RAG 集成了多模态数据和空间意识,在导航和解释任务中优于现有的RAG方法。文中还引入了一个新的数据集——Embodied-Experiences 数据集,以促进该领域的进一步研究。该系统的核心创新在于其构建和利用层次化空间记忆的能力,使机器人能够更有效地在不同的环境和查询类型中进行导航和交流。这项工作为开发基于语言的非参数记忆的通用机器人智能体奠定了基础。
原文链接:https://arxiv.org/abs/2409.18313