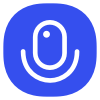
Sign up to save your podcasts
Or
Seventy3: 用NotebookLM将论文生成播客,让大家跟着AI一起进步。
今天的主题是:RL + Transformer = A General-Purpose Problem SolverSummary
This paper introduces an innovative approach called In-Context Reinforcement Learning (ICRL) that utilizes a pre-trained transformer model to solve problems, even those it hasn't seen before. The model, Llama 3.1 8B, is fine-tuned with reinforcement learning, enabling it to meta-learn and adapt to new environments with remarkable efficiency. The ICRL-trained transformer demonstrates the ability to combine learned skills, handle suboptimal training data, and adjust to changing environments, showcasing its potential as a general-purpose problem solver. The study assesses its performance on in-distribution and out-of-distribution environments, highlighting its ability to stitch together behaviors from its context and improve its solutions iteratively. The results indicate that ICRL holds promise for developing AI systems with human-like adaptability, although the ethical implications of autonomous agents are also considered and discussed. The work also reveals challenges related to exploration, suggesting potential avenues for future research to enhance the capabilities of ICRL-trained transformers.
该论文提出了一种创新方法——上下文强化学习(In-Context Reinforcement Learning, ICRL),该方法利用 预训练变换器模型 解决问题,包括此前未曾见过的问题。研究采用 Llama 3.1 8B 作为基础模型,并通过强化学习进行微调,使其具备元学习能力,从而能够高效适应新环境。实验表明,ICRL 训练的变换器能够整合已学技能、处理次优训练数据,并适应环境变化,展现出其作为通用问题求解器的潜力。研究评估了该模型在分布内(in-distribution)与分布外(out-of-distribution)环境中的表现,强调其能够基于上下文拼接行为(stitch together behaviors)并迭代优化解决方案。结果表明,ICRL 有望推动具备类人适应能力的人工智能系统的发展,同时研究也探讨了自主智能体的伦理影响。此外,研究揭示了 ICRL 在探索方面的挑战,并提出了未来研究方向,以进一步提升 ICRL 训练的变换器的能力。
原文链接:https://arxiv.org/abs/2501.14176
Seventy3: 用NotebookLM将论文生成播客,让大家跟着AI一起进步。
今天的主题是:RL + Transformer = A General-Purpose Problem SolverSummary
This paper introduces an innovative approach called In-Context Reinforcement Learning (ICRL) that utilizes a pre-trained transformer model to solve problems, even those it hasn't seen before. The model, Llama 3.1 8B, is fine-tuned with reinforcement learning, enabling it to meta-learn and adapt to new environments with remarkable efficiency. The ICRL-trained transformer demonstrates the ability to combine learned skills, handle suboptimal training data, and adjust to changing environments, showcasing its potential as a general-purpose problem solver. The study assesses its performance on in-distribution and out-of-distribution environments, highlighting its ability to stitch together behaviors from its context and improve its solutions iteratively. The results indicate that ICRL holds promise for developing AI systems with human-like adaptability, although the ethical implications of autonomous agents are also considered and discussed. The work also reveals challenges related to exploration, suggesting potential avenues for future research to enhance the capabilities of ICRL-trained transformers.
该论文提出了一种创新方法——上下文强化学习(In-Context Reinforcement Learning, ICRL),该方法利用 预训练变换器模型 解决问题,包括此前未曾见过的问题。研究采用 Llama 3.1 8B 作为基础模型,并通过强化学习进行微调,使其具备元学习能力,从而能够高效适应新环境。实验表明,ICRL 训练的变换器能够整合已学技能、处理次优训练数据,并适应环境变化,展现出其作为通用问题求解器的潜力。研究评估了该模型在分布内(in-distribution)与分布外(out-of-distribution)环境中的表现,强调其能够基于上下文拼接行为(stitch together behaviors)并迭代优化解决方案。结果表明,ICRL 有望推动具备类人适应能力的人工智能系统的发展,同时研究也探讨了自主智能体的伦理影响。此外,研究揭示了 ICRL 在探索方面的挑战,并提出了未来研究方向,以进一步提升 ICRL 训练的变换器的能力。
原文链接:https://arxiv.org/abs/2501.14176