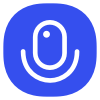
Sign up to save your podcasts
Or
Seventy3: 用NotebookLM将论文生成播客,让大家跟着AI一起进步。
今天的主题是:Return of the Encoder: Maximizing Parameter Efficiency for SLMsSummary
This paper challenges the current trend of using decoder-only architectures for language models, particularly for smaller language models (SLMs). It argues that encoder-decoder architectures offer superior efficiency and performance in resource-constrained environments, especially regarding latency and throughput on edge devices. The researchers introduce a knowledge distillation framework that allows encoder-decoder models to learn from larger decoder-only models while maintaining their architectural advantages. They also demonstrate the benefits of encoder-decoder models in vision-language tasks by integrating a vision encoder. Their findings suggest that focusing on architectural choices is crucial for creating efficient SLMs, especially for on-device deployment, rather than simply scaling down large models. They show that encoder-decoder models with knowledge distillation can outperform decoder-only models and reduce latency significantly.
该论文对当前以解码器(decoder-only)架构为主的语言模型趋势提出质疑,尤其针对小型语言模型(Small Language Models, SLMs)。研究表明,在资源受限环境(如边缘设备)中,编码器-解码器(encoder-decoder)架构在延迟和吞吐量方面表现更优,具备更高的效率和性能。为此,研究者提出了一种知识蒸馏(knowledge distillation)框架,使编码器-解码器模型能够从更大的解码器模型学习,同时保持其架构优势。此外,论文还通过集成视觉编码器(vision encoder),验证了编码器-解码器模型在视觉-语言任务中的优势。研究结果表明,优化架构选择比单纯缩小大模型规模更关键,尤其是在**端侧部署(on-device deployment)**的场景中。实验进一步证明,结合知识蒸馏的编码器-解码器模型不仅优于解码器模型,还能显著降低延迟。
原文链接:https://arxiv.org/abs/2501.16273
Seventy3: 用NotebookLM将论文生成播客,让大家跟着AI一起进步。
今天的主题是:Return of the Encoder: Maximizing Parameter Efficiency for SLMsSummary
This paper challenges the current trend of using decoder-only architectures for language models, particularly for smaller language models (SLMs). It argues that encoder-decoder architectures offer superior efficiency and performance in resource-constrained environments, especially regarding latency and throughput on edge devices. The researchers introduce a knowledge distillation framework that allows encoder-decoder models to learn from larger decoder-only models while maintaining their architectural advantages. They also demonstrate the benefits of encoder-decoder models in vision-language tasks by integrating a vision encoder. Their findings suggest that focusing on architectural choices is crucial for creating efficient SLMs, especially for on-device deployment, rather than simply scaling down large models. They show that encoder-decoder models with knowledge distillation can outperform decoder-only models and reduce latency significantly.
该论文对当前以解码器(decoder-only)架构为主的语言模型趋势提出质疑,尤其针对小型语言模型(Small Language Models, SLMs)。研究表明,在资源受限环境(如边缘设备)中,编码器-解码器(encoder-decoder)架构在延迟和吞吐量方面表现更优,具备更高的效率和性能。为此,研究者提出了一种知识蒸馏(knowledge distillation)框架,使编码器-解码器模型能够从更大的解码器模型学习,同时保持其架构优势。此外,论文还通过集成视觉编码器(vision encoder),验证了编码器-解码器模型在视觉-语言任务中的优势。研究结果表明,优化架构选择比单纯缩小大模型规模更关键,尤其是在**端侧部署(on-device deployment)**的场景中。实验进一步证明,结合知识蒸馏的编码器-解码器模型不仅优于解码器模型,还能显著降低延迟。
原文链接:https://arxiv.org/abs/2501.16273