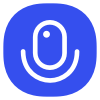
Sign up to save your podcasts
Or
Seventy3: 用NotebookLM将论文生成播客,让大家跟着AI一起进步。
今天的主题是:Thoughts Are All Over the Place: On the Underthinking of o1-Like LLMsSummary
This research investigates "underthinking" in large language models (LLMs), where models prematurely switch between reasoning strategies on complex tasks. The authors found that this frequent thought-switching correlates with incorrect answers and propose a metric to quantify this inefficiency. To address this, they introduce a "thought switching penalty" (TIP) during decoding, discouraging early transitions between reasoning paths. Experiments show that TIP improves accuracy without fine-tuning the model. The study contributes to understanding and mitigating reasoning inefficiencies in LLMs, enhancing their problem-solving capabilities. The authors analyze prior work in reasoning with LLMs, as well as manipulation of decoding penalties.
本研究探讨了大型语言模型(LLM)的“思维不足”问题,即在处理复杂任务时,模型过早切换推理策略。作者发现,这种频繁的思维切换与错误答案存在相关性,并提出了一种量化该低效性的指标。为了解决这一问题,研究在解码过程中引入了“思维切换惩罚”(TIP),以抑制推理路径的过早转换。实验表明,TIP 在无需微调模型的情况下提高了准确率。本研究有助于理解并缓解 LLM 的推理低效性,增强其问题解决能力。作者还分析了 LLM 推理相关的先前研究以及解码惩罚的调整方法。
原文链接:https://arxiv.org/abs/2501.18585
Seventy3: 用NotebookLM将论文生成播客,让大家跟着AI一起进步。
今天的主题是:Thoughts Are All Over the Place: On the Underthinking of o1-Like LLMsSummary
This research investigates "underthinking" in large language models (LLMs), where models prematurely switch between reasoning strategies on complex tasks. The authors found that this frequent thought-switching correlates with incorrect answers and propose a metric to quantify this inefficiency. To address this, they introduce a "thought switching penalty" (TIP) during decoding, discouraging early transitions between reasoning paths. Experiments show that TIP improves accuracy without fine-tuning the model. The study contributes to understanding and mitigating reasoning inefficiencies in LLMs, enhancing their problem-solving capabilities. The authors analyze prior work in reasoning with LLMs, as well as manipulation of decoding penalties.
本研究探讨了大型语言模型(LLM)的“思维不足”问题,即在处理复杂任务时,模型过早切换推理策略。作者发现,这种频繁的思维切换与错误答案存在相关性,并提出了一种量化该低效性的指标。为了解决这一问题,研究在解码过程中引入了“思维切换惩罚”(TIP),以抑制推理路径的过早转换。实验表明,TIP 在无需微调模型的情况下提高了准确率。本研究有助于理解并缓解 LLM 的推理低效性,增强其问题解决能力。作者还分析了 LLM 推理相关的先前研究以及解码惩罚的调整方法。
原文链接:https://arxiv.org/abs/2501.18585