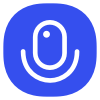
Sign up to save your podcasts
Or
Seventy3: 用NotebookLM将论文生成播客,让大家跟着AI一起进步。
今天的主题是:GCBF+: A Neural Graph Control Barrier Function Framework for Distributed Safe Multi-Agent ControlSummary
This research introduces a novel framework, GCBF+, for safe and scalable control of multi-agent systems using Graph Control Barrier Functions (GCBFs). The framework employs graph neural networks to learn GCBFs and distributed control policies, enabling agents to avoid collisions and reach goals using only local information. A key contribution is a theoretical result proving that a single GCBF can guarantee safety for multi-agent systems of arbitrary size, even when trained on smaller groups. Experimental results, including hardware tests with Crazyflie drones, demonstrate GCBF+'s superior performance compared to existing methods, especially in complex, nonlinear environments. The framework addresses limitations of prior approaches by incorporating actuation limits and using a new loss function that avoids the safety versus goal-reaching trade-off typical in reinforcement learning. The proposed GCBF+ method is shown to be robust to a large range of its hyper-parameters for successful goal reaching and safety maintenance.
本研究提出了一种新颖的框架 GCBF+,利用图控制屏障函数(GCBF)实现多智能体系统的安全可扩展控制。该框架采用图神经网络来学习 GCBF 和分布式控制策略,使智能体仅依赖局部信息即可避障并到达目标。研究的核心贡献之一是理论证明:即使仅在小规模智能体群体上训练,一个单一的 GCBF 也能确保任意规模的多智能体系统的安全。实验结果(包括 Crazyflie 无人机的硬件测试)表明,GCBF+ 在复杂非线性环境中相较现有方法表现更优。该框架通过引入执行限制并采用新的损失函数,克服了以往方法的局限性,避免了强化学习中常见的“安全性与目标达成之间的权衡”问题。研究还表明,GCBF+ 在较广泛的超参数范围内均能保持目标达成和系统安全的鲁棒性。
原文链接:https://arxiv.org/abs/2401.14554
Seventy3: 用NotebookLM将论文生成播客,让大家跟着AI一起进步。
今天的主题是:GCBF+: A Neural Graph Control Barrier Function Framework for Distributed Safe Multi-Agent ControlSummary
This research introduces a novel framework, GCBF+, for safe and scalable control of multi-agent systems using Graph Control Barrier Functions (GCBFs). The framework employs graph neural networks to learn GCBFs and distributed control policies, enabling agents to avoid collisions and reach goals using only local information. A key contribution is a theoretical result proving that a single GCBF can guarantee safety for multi-agent systems of arbitrary size, even when trained on smaller groups. Experimental results, including hardware tests with Crazyflie drones, demonstrate GCBF+'s superior performance compared to existing methods, especially in complex, nonlinear environments. The framework addresses limitations of prior approaches by incorporating actuation limits and using a new loss function that avoids the safety versus goal-reaching trade-off typical in reinforcement learning. The proposed GCBF+ method is shown to be robust to a large range of its hyper-parameters for successful goal reaching and safety maintenance.
本研究提出了一种新颖的框架 GCBF+,利用图控制屏障函数(GCBF)实现多智能体系统的安全可扩展控制。该框架采用图神经网络来学习 GCBF 和分布式控制策略,使智能体仅依赖局部信息即可避障并到达目标。研究的核心贡献之一是理论证明:即使仅在小规模智能体群体上训练,一个单一的 GCBF 也能确保任意规模的多智能体系统的安全。实验结果(包括 Crazyflie 无人机的硬件测试)表明,GCBF+ 在复杂非线性环境中相较现有方法表现更优。该框架通过引入执行限制并采用新的损失函数,克服了以往方法的局限性,避免了强化学习中常见的“安全性与目标达成之间的权衡”问题。研究还表明,GCBF+ 在较广泛的超参数范围内均能保持目标达成和系统安全的鲁棒性。
原文链接:https://arxiv.org/abs/2401.14554