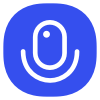
Sign up to save your podcasts
Or
Seventy3: 用NotebookLM将论文生成播客,让大家跟着AI一起进步。
今天的主题是:Demystifying Long Chain-of-Thought Reasoning in LLMsSummary
This paper investigates how large language models (LLMs) achieve long chain-of-thought (CoT) reasoning, which involves extended, step-by-step thought processes for complex tasks. The authors explore the roles of supervised fine-tuning (SFT) and reinforcement learning (RL) in enabling this capability. Key findings highlight that while SFT on long CoT data improves performance and facilitates better RL, carefully designed reward functions are crucial for stable CoT length and enhanced reasoning. The study also examines the use of noisy web data for training and nuances in analyzing emergent reasoning behaviors during RL from base models. Ultimately, the research offers practical insights for optimizing training strategies to bolster sophisticated reasoning in LLMs.
本文探讨了大型语言模型(LLMs)如何实现长链式思维(CoT)推理,即在复杂任务中执行逐步、扩展的思考过程。作者研究了监督微调(SFT)和强化学习(RL)在提升这一能力中的作用。关键发现包括:虽然在长 CoT 数据上进行 SFT 可提高性能并优化 RL 训练,但精心设计的奖励函数对于稳定 CoT 长度和增强推理能力至关重要。此外,研究还分析了带噪声的网页数据用于训练的影响,以及在 RL 过程中基于基础模型解析涌现推理行为的细微差别。最终,该研究提供了优化训练策略的实用见解,以提升 LLMs 的高级推理能力。
原文链接:https://arxiv.org/abs/2502.03373
####🥚####彩####蛋####🥚####
本博客从24年10月2日开启,已更新半年,借助NotebookLM的能力进行论文解读,专注人工智能、大模型、机器人算法的论文,也有几十个听友。本人是一介书生,现计划建立微信群,平时可以聊聊技术,聊聊生活。希望博客可以继续更新下去!
进群添加微信小助手:seventy3_podcast
备注:小宇宙
####🥚####彩####蛋####🥚####
Seventy3: 用NotebookLM将论文生成播客,让大家跟着AI一起进步。
今天的主题是:Demystifying Long Chain-of-Thought Reasoning in LLMsSummary
This paper investigates how large language models (LLMs) achieve long chain-of-thought (CoT) reasoning, which involves extended, step-by-step thought processes for complex tasks. The authors explore the roles of supervised fine-tuning (SFT) and reinforcement learning (RL) in enabling this capability. Key findings highlight that while SFT on long CoT data improves performance and facilitates better RL, carefully designed reward functions are crucial for stable CoT length and enhanced reasoning. The study also examines the use of noisy web data for training and nuances in analyzing emergent reasoning behaviors during RL from base models. Ultimately, the research offers practical insights for optimizing training strategies to bolster sophisticated reasoning in LLMs.
本文探讨了大型语言模型(LLMs)如何实现长链式思维(CoT)推理,即在复杂任务中执行逐步、扩展的思考过程。作者研究了监督微调(SFT)和强化学习(RL)在提升这一能力中的作用。关键发现包括:虽然在长 CoT 数据上进行 SFT 可提高性能并优化 RL 训练,但精心设计的奖励函数对于稳定 CoT 长度和增强推理能力至关重要。此外,研究还分析了带噪声的网页数据用于训练的影响,以及在 RL 过程中基于基础模型解析涌现推理行为的细微差别。最终,该研究提供了优化训练策略的实用见解,以提升 LLMs 的高级推理能力。
原文链接:https://arxiv.org/abs/2502.03373
####🥚####彩####蛋####🥚####
本博客从24年10月2日开启,已更新半年,借助NotebookLM的能力进行论文解读,专注人工智能、大模型、机器人算法的论文,也有几十个听友。本人是一介书生,现计划建立微信群,平时可以聊聊技术,聊聊生活。希望博客可以继续更新下去!
进群添加微信小助手:seventy3_podcast
备注:小宇宙
####🥚####彩####蛋####🥚####