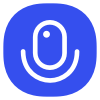
Sign up to save your podcasts
Or
Seventy3:借助NotebookLM的能力进行论文解读,专注人工智能、大模型、机器人算法方向,让大家跟着AI一起进步。
进群添加小助手微信:seventy3_podcast
备注:小宇宙
今天的主题是:Rethinking External Slow-Thinking: From Snowball Errors to Probability of Correct ReasoningSummary
This paper examines "slow-thinking" in large language models (LLMs), where increased computation time enhances reasoning. It theoretically analyzes how errors accumulate during LLM reasoning, termed "snowball errors," linking them to the decreasing probability of correct reasoning through information theory. The research proposes that external slow-thinking methods, which involve expanding the search for solutions, primarily work by mitigating these error probabilities. A comparative analysis of various slow-thinking approaches, including Best-of-N and Monte Carlo Tree Search, suggests their effectiveness hinges more on the reliability of evaluation mechanisms and overall computational cost than the specific algorithmic framework. Ultimately, the study advocates for focusing on improving reward functions and core reasoning capabilities for better slow-thinking strategies.
本文研究了大型语言模型(LLMs)中的**“慢思考”(slow-thinking)现象,即增加计算时间如何提升推理能力**。作者从理论角度分析了 LLM 推理过程中错误的积累机制,并将其定义为**“滚雪球错误”(snowball errors),借助信息论揭示了正确推理概率随推理深度降低的趋势**。研究提出,外部慢思考方法(如扩展解空间搜索)主要通过降低错误概率来提升推理质量。对比分析了多种慢思考方法,包括Best-of-N 和 蒙特卡洛树搜索(MCTS),结果表明,其有效性更多取决于评估机制的可靠性和计算成本,而非具体算法框架。最终,研究强调优化奖励函数和核心推理能力的重要性,以改进慢思考策略。
原文链接:https://arxiv.org/abs/2501.15602
Seventy3:借助NotebookLM的能力进行论文解读,专注人工智能、大模型、机器人算法方向,让大家跟着AI一起进步。
进群添加小助手微信:seventy3_podcast
备注:小宇宙
今天的主题是:Rethinking External Slow-Thinking: From Snowball Errors to Probability of Correct ReasoningSummary
This paper examines "slow-thinking" in large language models (LLMs), where increased computation time enhances reasoning. It theoretically analyzes how errors accumulate during LLM reasoning, termed "snowball errors," linking them to the decreasing probability of correct reasoning through information theory. The research proposes that external slow-thinking methods, which involve expanding the search for solutions, primarily work by mitigating these error probabilities. A comparative analysis of various slow-thinking approaches, including Best-of-N and Monte Carlo Tree Search, suggests their effectiveness hinges more on the reliability of evaluation mechanisms and overall computational cost than the specific algorithmic framework. Ultimately, the study advocates for focusing on improving reward functions and core reasoning capabilities for better slow-thinking strategies.
本文研究了大型语言模型(LLMs)中的**“慢思考”(slow-thinking)现象,即增加计算时间如何提升推理能力**。作者从理论角度分析了 LLM 推理过程中错误的积累机制,并将其定义为**“滚雪球错误”(snowball errors),借助信息论揭示了正确推理概率随推理深度降低的趋势**。研究提出,外部慢思考方法(如扩展解空间搜索)主要通过降低错误概率来提升推理质量。对比分析了多种慢思考方法,包括Best-of-N 和 蒙特卡洛树搜索(MCTS),结果表明,其有效性更多取决于评估机制的可靠性和计算成本,而非具体算法框架。最终,研究强调优化奖励函数和核心推理能力的重要性,以改进慢思考策略。
原文链接:https://arxiv.org/abs/2501.15602