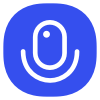
Sign up to save your podcasts
Or
Seventy3:借助NotebookLM的能力进行论文解读,专注人工智能、大模型、机器人算法方向,让大家跟着AI一起进步。
进群添加小助手微信:seventy3_podcast
备注:小宇宙
今天的主题是:CoAT: Chain-of-Associated-Thoughts Framework for Enhancing Large Language Models ReasoningSummary
The provided research paper introduces CoAT, a novel framework designed to enhance the reasoning capabilities of large language models (LLMs). Inspired by human cognition, CoAT integrates Monte Carlo Tree Search (MCTS) for structured exploration of reasoning paths with an associative memory mechanism that dynamically incorporates new information. This synergy allows LLMs to revisit prior inferences and adapt to evolving data, leading to more accurate, coherent, and diverse outputs, as validated through extensive experiments on generative and reasoning tasks, including comparisons with other knowledge-augmented methods and fine-tuned models. The paper details the architecture and implementation of CoAT, including its associative memory and optimized MCTS, and presents both qualitative and quantitative evidence of its superior performance across various NLP and code generation benchmarks.
本文提出了 CoAT,一个创新框架,旨在增强大型语言模型(LLMs)的推理能力。受人类认知启发,CoAT 结合了蒙特卡洛树搜索(MCTS),用于结构化探索推理路径,并引入联想记忆机制,动态整合新信息。这种协同作用使 LLMs 能够回溯先前推理并适应不断变化的数据,从而生成更准确、连贯、多样的输出。通过广泛实验,包括与其他知识增强方法及微调模型的对比,研究验证了 CoAT 在生成与推理任务中的有效性。论文详细介绍了 CoAT 的架构与实现,包括联想记忆模块和优化的 MCTS 算法,并在多个NLP 和代码生成基准上提供了定性和定量证据,证明其卓越性能。
原文链接:https://arxiv.org/abs/2502.02390
Seventy3:借助NotebookLM的能力进行论文解读,专注人工智能、大模型、机器人算法方向,让大家跟着AI一起进步。
进群添加小助手微信:seventy3_podcast
备注:小宇宙
今天的主题是:CoAT: Chain-of-Associated-Thoughts Framework for Enhancing Large Language Models ReasoningSummary
The provided research paper introduces CoAT, a novel framework designed to enhance the reasoning capabilities of large language models (LLMs). Inspired by human cognition, CoAT integrates Monte Carlo Tree Search (MCTS) for structured exploration of reasoning paths with an associative memory mechanism that dynamically incorporates new information. This synergy allows LLMs to revisit prior inferences and adapt to evolving data, leading to more accurate, coherent, and diverse outputs, as validated through extensive experiments on generative and reasoning tasks, including comparisons with other knowledge-augmented methods and fine-tuned models. The paper details the architecture and implementation of CoAT, including its associative memory and optimized MCTS, and presents both qualitative and quantitative evidence of its superior performance across various NLP and code generation benchmarks.
本文提出了 CoAT,一个创新框架,旨在增强大型语言模型(LLMs)的推理能力。受人类认知启发,CoAT 结合了蒙特卡洛树搜索(MCTS),用于结构化探索推理路径,并引入联想记忆机制,动态整合新信息。这种协同作用使 LLMs 能够回溯先前推理并适应不断变化的数据,从而生成更准确、连贯、多样的输出。通过广泛实验,包括与其他知识增强方法及微调模型的对比,研究验证了 CoAT 在生成与推理任务中的有效性。论文详细介绍了 CoAT 的架构与实现,包括联想记忆模块和优化的 MCTS 算法,并在多个NLP 和代码生成基准上提供了定性和定量证据,证明其卓越性能。
原文链接:https://arxiv.org/abs/2502.02390