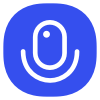
Sign up to save your podcasts
Or
Seventy3:借助NotebookLM的能力进行论文解读,专注人工智能、大模型、机器人算法方向,让大家跟着AI一起进步。
进群添加小助手微信:seventy3_podcast
备注:小宇宙
今天的主题是:Rethinking Mixture-of-Agents: Is Mixing Different Large Language Models Beneficial?Summary
This paper investigates Mixture-of-Agents (MoA), a method that combines outputs from different large language models (LLMs), and introduces Self-MoA, which aggregates multiple outputs from a single top-performing LLM. Surprisingly, Self-MoA often outperforms standard MoA across various benchmarks by better balancing the trade-off between output quality and diversity. The authors further explore this quality-diversity relationship and present Self-MoA-Seq, a sequential version for handling large numbers of outputs with limited context windows, suggesting that focusing on the strength of individual models can be more beneficial than solely pursuing diversity in LLM ensembles.
本文研究了 Mixture-of-Agents(MoA) 方法,该方法通过组合不同大语言模型(LLMs)的输出来提升性能,并提出了 Self-MoA,即从单个顶级 LLM 生成多个输出并进行聚合。令人意外的是,Self-MoA 在多个基准测试上往往优于传统 MoA,因为它能更好地平衡输出质量与多样性之间的权衡。作者进一步探讨了这一质量-多样性关系,并提出了 Self-MoA-Seq,一种适用于有限上下文窗口的大规模输出处理的序列化版本。研究表明,与单纯追求 LLM 集成的多样性相比,充分利用单个模型的优势可能更加有效。
原文链接:https://arxiv.org/abs/2502.00674
Seventy3:借助NotebookLM的能力进行论文解读,专注人工智能、大模型、机器人算法方向,让大家跟着AI一起进步。
进群添加小助手微信:seventy3_podcast
备注:小宇宙
今天的主题是:Rethinking Mixture-of-Agents: Is Mixing Different Large Language Models Beneficial?Summary
This paper investigates Mixture-of-Agents (MoA), a method that combines outputs from different large language models (LLMs), and introduces Self-MoA, which aggregates multiple outputs from a single top-performing LLM. Surprisingly, Self-MoA often outperforms standard MoA across various benchmarks by better balancing the trade-off between output quality and diversity. The authors further explore this quality-diversity relationship and present Self-MoA-Seq, a sequential version for handling large numbers of outputs with limited context windows, suggesting that focusing on the strength of individual models can be more beneficial than solely pursuing diversity in LLM ensembles.
本文研究了 Mixture-of-Agents(MoA) 方法,该方法通过组合不同大语言模型(LLMs)的输出来提升性能,并提出了 Self-MoA,即从单个顶级 LLM 生成多个输出并进行聚合。令人意外的是,Self-MoA 在多个基准测试上往往优于传统 MoA,因为它能更好地平衡输出质量与多样性之间的权衡。作者进一步探讨了这一质量-多样性关系,并提出了 Self-MoA-Seq,一种适用于有限上下文窗口的大规模输出处理的序列化版本。研究表明,与单纯追求 LLM 集成的多样性相比,充分利用单个模型的优势可能更加有效。
原文链接:https://arxiv.org/abs/2502.00674