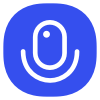
Sign up to save your podcasts
Or
Seventy3:借助NotebookLM的能力进行论文解读,专注人工智能、大模型、机器人算法方向,让大家跟着AI一起进步。
进群添加小助手微信:seventy3_podcast
备注:小宇宙
今天的主题是:Advancing Reasoning in Large Language Models: Promising Methods and ApproachesSummary
This document provides a survey of techniques aimed at improving the reasoning abilities of Large Language Models (LLMs), which often struggle with complex logical tasks despite their proficiency in natural language processing. The author categorizes these methods into prompting strategies, such as chain-of-thought reasoning, architectural innovations, like retrieval-augmented generation, and learning paradigms, including fine-tuning and reinforcement learning. The survey also discusses evaluation benchmarks used to assess reasoning in LLMs and highlights ongoing challenges such as hallucinations and the need for better generalization. Ultimately, the paper aims to synthesize recent advancements and offer insights into future research directions for developing more capable reasoning-augmented LLMs, even mentioning the recently released DeepSeek-R1 as an example of progress in this area.
本文对旨在提升大型语言模型(LLMs)推理能力的技术进行了综述,尽管 LLMs 在自然语言处理方面表现出色,但在复杂的逻辑任务中往往存在困难。作者将这些方法分为几类:提示策略(如链式推理)、架构创新(如检索增强生成)和学习范式(包括微调和强化学习)。综述还讨论了用于评估 LLM 推理能力的基准测试,并强调了当前面临的挑战,如幻觉问题和对更好泛化能力的需求。最终,本文旨在综合近期的进展,并提供关于开发更强推理能力的 LLMs的未来研究方向的见解,甚至提到最近发布的 DeepSeek-R1,作为该领域进展的例子。
原文链接:https://arxiv.org/abs/2502.03671
Seventy3:借助NotebookLM的能力进行论文解读,专注人工智能、大模型、机器人算法方向,让大家跟着AI一起进步。
进群添加小助手微信:seventy3_podcast
备注:小宇宙
今天的主题是:Advancing Reasoning in Large Language Models: Promising Methods and ApproachesSummary
This document provides a survey of techniques aimed at improving the reasoning abilities of Large Language Models (LLMs), which often struggle with complex logical tasks despite their proficiency in natural language processing. The author categorizes these methods into prompting strategies, such as chain-of-thought reasoning, architectural innovations, like retrieval-augmented generation, and learning paradigms, including fine-tuning and reinforcement learning. The survey also discusses evaluation benchmarks used to assess reasoning in LLMs and highlights ongoing challenges such as hallucinations and the need for better generalization. Ultimately, the paper aims to synthesize recent advancements and offer insights into future research directions for developing more capable reasoning-augmented LLMs, even mentioning the recently released DeepSeek-R1 as an example of progress in this area.
本文对旨在提升大型语言模型(LLMs)推理能力的技术进行了综述,尽管 LLMs 在自然语言处理方面表现出色,但在复杂的逻辑任务中往往存在困难。作者将这些方法分为几类:提示策略(如链式推理)、架构创新(如检索增强生成)和学习范式(包括微调和强化学习)。综述还讨论了用于评估 LLM 推理能力的基准测试,并强调了当前面临的挑战,如幻觉问题和对更好泛化能力的需求。最终,本文旨在综合近期的进展,并提供关于开发更强推理能力的 LLMs的未来研究方向的见解,甚至提到最近发布的 DeepSeek-R1,作为该领域进展的例子。
原文链接:https://arxiv.org/abs/2502.03671