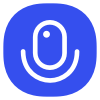
Sign up to save your podcasts
Or
Seventy3:借助NotebookLM的能力进行论文解读,专注人工智能、大模型、机器人算法方向,让大家跟着AI一起进步。
进群添加小助手微信:seventy3_podcast
备注:小宇宙
今天的主题是:Value-Based Deep RL Scales PredictablySummary
This research investigates the scaling properties of value-based deep reinforcement learning methods. The authors demonstrate that despite common beliefs, the performance of these methods can be predicted as computational resources and training data increase. They establish predictable relationships between key hyperparameters like batch size and learning rate, and the updates-to-data ratio. Furthermore, the study reveals a predictable Pareto frontier between data and compute requirements to achieve specific performance levels. This allows for the extrapolation of resource needs and optimal hyperparameter settings from small-scale experiments to larger, more demanding scenarios. Ultimately, the work challenges the notion that value-based RL scales unpredictably, offering insights for more efficient resource allocation in advanced RL applications.
这项研究探讨了基于价值的深度强化学习方法的可扩展性特征。作者们展示了,尽管普遍认为这些方法在扩展时表现难以预测,但其实它们的性能是可以随着计算资源和训练数据的增加而进行预测的。他们建立了关键超参数(如批量大小、学习率)与“更新次数与数据量”之间的可预测关系。此外,研究还揭示了在达到特定性能水平时,数据需求与计算资源之间存在一个可预测的帕累托前沿。这使得可以通过小规模实验外推出在更大、更复杂场景下的资源需求和最优超参数设置。最终,该研究挑战了“基于价值的强化学习在扩展时表现不可预测”的观点,为在高级强化学习应用中实现更高效的资源分配提供了新见解。
原文链接:https://arxiv.org/abs/2502.04327
Seventy3:借助NotebookLM的能力进行论文解读,专注人工智能、大模型、机器人算法方向,让大家跟着AI一起进步。
进群添加小助手微信:seventy3_podcast
备注:小宇宙
今天的主题是:Value-Based Deep RL Scales PredictablySummary
This research investigates the scaling properties of value-based deep reinforcement learning methods. The authors demonstrate that despite common beliefs, the performance of these methods can be predicted as computational resources and training data increase. They establish predictable relationships between key hyperparameters like batch size and learning rate, and the updates-to-data ratio. Furthermore, the study reveals a predictable Pareto frontier between data and compute requirements to achieve specific performance levels. This allows for the extrapolation of resource needs and optimal hyperparameter settings from small-scale experiments to larger, more demanding scenarios. Ultimately, the work challenges the notion that value-based RL scales unpredictably, offering insights for more efficient resource allocation in advanced RL applications.
这项研究探讨了基于价值的深度强化学习方法的可扩展性特征。作者们展示了,尽管普遍认为这些方法在扩展时表现难以预测,但其实它们的性能是可以随着计算资源和训练数据的增加而进行预测的。他们建立了关键超参数(如批量大小、学习率)与“更新次数与数据量”之间的可预测关系。此外,研究还揭示了在达到特定性能水平时,数据需求与计算资源之间存在一个可预测的帕累托前沿。这使得可以通过小规模实验外推出在更大、更复杂场景下的资源需求和最优超参数设置。最终,该研究挑战了“基于价值的强化学习在扩展时表现不可预测”的观点,为在高级强化学习应用中实现更高效的资源分配提供了新见解。
原文链接:https://arxiv.org/abs/2502.04327