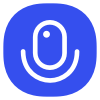
Sign up to save your podcasts
Or
Seventy3:借助NotebookLM的能力进行论文解读,专注人工智能、大模型、机器人算法方向,让大家跟着AI一起进步。
进群添加小助手微信:seventy3_podcast
备注:小宇宙
今天的主题是:On Limitations of the Transformer ArchitectureSummary
This paper explores theoretical limitations of the Transformer architecture, a cornerstone of large language models. Through the lens of Communication Complexity, the authors demonstrate that a single Transformer layer struggles with function composition when dealing with sufficiently large data domains, a weakness empirically evident even with smaller datasets. Furthermore, by employing Computational Complexity theory, the paper argues that multi-layer Transformers inherently face difficulties with tasks requiring sequential composition and logical reasoning due to memory constraints, suggesting a fundamental incompatibility unless certain complexity conjectures are false. These findings provide potential explanations for the hallucination and compositionality issues observed in large language models.
这篇论文探讨了Transformer架构在理论上的局限性,Transformer是大型语言模型的基石。作者通过通信复杂性的视角表明,当数据域足够大时,单层Transformer在处理函数组合方面存在困难,这一弱点在较小数据集上也有经验性证据可循。此外,论文运用计算复杂性理论进一步指出,多层Transformer在需要顺序组合和逻辑推理的任务中也面临固有的挑战,其根本原因在于内存限制——除非某些复杂性猜想被推翻,否则这种不适应是理论上不可避免的。这些发现为大型语言模型中出现的幻觉现象和组合性问题提供了可能的理论解释。
原文链接:https://arxiv.org/abs/2402.08164
Seventy3:借助NotebookLM的能力进行论文解读,专注人工智能、大模型、机器人算法方向,让大家跟着AI一起进步。
进群添加小助手微信:seventy3_podcast
备注:小宇宙
今天的主题是:On Limitations of the Transformer ArchitectureSummary
This paper explores theoretical limitations of the Transformer architecture, a cornerstone of large language models. Through the lens of Communication Complexity, the authors demonstrate that a single Transformer layer struggles with function composition when dealing with sufficiently large data domains, a weakness empirically evident even with smaller datasets. Furthermore, by employing Computational Complexity theory, the paper argues that multi-layer Transformers inherently face difficulties with tasks requiring sequential composition and logical reasoning due to memory constraints, suggesting a fundamental incompatibility unless certain complexity conjectures are false. These findings provide potential explanations for the hallucination and compositionality issues observed in large language models.
这篇论文探讨了Transformer架构在理论上的局限性,Transformer是大型语言模型的基石。作者通过通信复杂性的视角表明,当数据域足够大时,单层Transformer在处理函数组合方面存在困难,这一弱点在较小数据集上也有经验性证据可循。此外,论文运用计算复杂性理论进一步指出,多层Transformer在需要顺序组合和逻辑推理的任务中也面临固有的挑战,其根本原因在于内存限制——除非某些复杂性猜想被推翻,否则这种不适应是理论上不可避免的。这些发现为大型语言模型中出现的幻觉现象和组合性问题提供了可能的理论解释。
原文链接:https://arxiv.org/abs/2402.08164