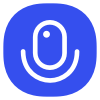
Sign up to save your podcasts
Or
Seventy3:借助NotebookLM的能力进行论文解读,专注人工智能、大模型、机器人算法方向,让大家跟着AI一起进步。
进群添加小助手微信:seventy3_podcast
备注:小宇宙
今天的主题是:LM2: Large Memory ModelsSummary
This paper introduces the Large Memory Model (LM2), a novel Transformer architecture enhanced with an auxiliary memory module to improve performance on tasks requiring long context and complex reasoning. The LM2's memory component stores and retrieves contextual information, interacting with input tokens via cross attention and updating through gating mechanisms, while preserving the original Transformer information flow. Experiments on the BABILong benchmark demonstrate LM2's significant outperformance compared to memory-augmented and baseline models, especially in multi-hop inference and question-answering. Furthermore, LM2 maintains strong performance on general tasks as evidenced by results on the MMLU dataset, indicating that the integration of the memory module does not hinder overall capabilities. The research highlights the importance of explicit memory mechanisms for enhancing Transformer architectures.
这篇论文提出了大型记忆模型(LM2),这是一种新颖的Transformer架构,结合了辅助记忆模块,以提升在长上下文依赖和复杂推理任务中的表现。LM2的记忆组件能够存储并检索上下文信息,通过交叉注意力机制与输入token交互,并通过门控机制进行更新,同时保留了原始Transformer的信息流结构。
在BABILong基准测试上的实验表明,LM2在表现上显著优于其他带记忆增强的模型和基线模型,特别是在多跳推理和问答任务中表现尤为突出。此外,LM2在通用任务中也保持了强劲性能,如在MMLU数据集上的测试结果所示,证明引入记忆模块并未削弱模型的整体能力。
本研究强调了显式记忆机制在提升Transformer架构能力方面的重要性。
原文链接:https://arxiv.org/abs/2502.06049
Seventy3:借助NotebookLM的能力进行论文解读,专注人工智能、大模型、机器人算法方向,让大家跟着AI一起进步。
进群添加小助手微信:seventy3_podcast
备注:小宇宙
今天的主题是:LM2: Large Memory ModelsSummary
This paper introduces the Large Memory Model (LM2), a novel Transformer architecture enhanced with an auxiliary memory module to improve performance on tasks requiring long context and complex reasoning. The LM2's memory component stores and retrieves contextual information, interacting with input tokens via cross attention and updating through gating mechanisms, while preserving the original Transformer information flow. Experiments on the BABILong benchmark demonstrate LM2's significant outperformance compared to memory-augmented and baseline models, especially in multi-hop inference and question-answering. Furthermore, LM2 maintains strong performance on general tasks as evidenced by results on the MMLU dataset, indicating that the integration of the memory module does not hinder overall capabilities. The research highlights the importance of explicit memory mechanisms for enhancing Transformer architectures.
这篇论文提出了大型记忆模型(LM2),这是一种新颖的Transformer架构,结合了辅助记忆模块,以提升在长上下文依赖和复杂推理任务中的表现。LM2的记忆组件能够存储并检索上下文信息,通过交叉注意力机制与输入token交互,并通过门控机制进行更新,同时保留了原始Transformer的信息流结构。
在BABILong基准测试上的实验表明,LM2在表现上显著优于其他带记忆增强的模型和基线模型,特别是在多跳推理和问答任务中表现尤为突出。此外,LM2在通用任务中也保持了强劲性能,如在MMLU数据集上的测试结果所示,证明引入记忆模块并未削弱模型的整体能力。
本研究强调了显式记忆机制在提升Transformer架构能力方面的重要性。
原文链接:https://arxiv.org/abs/2502.06049