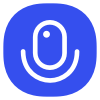
Sign up to save your podcasts
Or
Seventy3:借助NotebookLM的能力进行论文解读,专注人工智能、大模型、机器人算法方向,让大家跟着AI一起进步。
进群添加小助手微信:seventy3_podcast
备注:小宇宙
今天的主题是:Scaling up Test-Time Compute with Latent Reasoning: A Recurrent Depth ApproachSummary
This paper introduces a novel language model architecture that enhances reasoning by iteratively processing information in a latent space rather than solely generating more tokens. This "recurrent depth" approach allows the model to increase its computational effort at test time without needing specialized training data or long context windows, potentially capturing nuanced reasoning. The authors scaled a proof-of-concept model, demonstrating performance gains on reasoning benchmarks by increasing test-time computation. Additionally, this architecture naturally supports features like adaptive compute and KV-cache sharing, suggesting a promising direction for more efficient and powerful language models.
这篇论文介绍了一种新型的语言模型架构,通过在潜在空间中迭代处理信息来增强推理能力,而不仅仅是生成更多的token。这个“递归深度”方法使得模型在测试时能够增加计算力度,而不需要专门的训练数据或长时间的上下文窗口,从而可能捕捉到更细微的推理过程。
作者通过扩展一个概念验证模型,证明了通过增加测试时计算量,在推理基准测试上能够获得性能提升。此外,这种架构自然支持自适应计算和KV缓存共享等特性,暗示着它在实现更高效、更强大的语言模型方面具有很大的潜力。
原文链接:https://arxiv.org/abs/2502.05171
Seventy3:借助NotebookLM的能力进行论文解读,专注人工智能、大模型、机器人算法方向,让大家跟着AI一起进步。
进群添加小助手微信:seventy3_podcast
备注:小宇宙
今天的主题是:Scaling up Test-Time Compute with Latent Reasoning: A Recurrent Depth ApproachSummary
This paper introduces a novel language model architecture that enhances reasoning by iteratively processing information in a latent space rather than solely generating more tokens. This "recurrent depth" approach allows the model to increase its computational effort at test time without needing specialized training data or long context windows, potentially capturing nuanced reasoning. The authors scaled a proof-of-concept model, demonstrating performance gains on reasoning benchmarks by increasing test-time computation. Additionally, this architecture naturally supports features like adaptive compute and KV-cache sharing, suggesting a promising direction for more efficient and powerful language models.
这篇论文介绍了一种新型的语言模型架构,通过在潜在空间中迭代处理信息来增强推理能力,而不仅仅是生成更多的token。这个“递归深度”方法使得模型在测试时能够增加计算力度,而不需要专门的训练数据或长时间的上下文窗口,从而可能捕捉到更细微的推理过程。
作者通过扩展一个概念验证模型,证明了通过增加测试时计算量,在推理基准测试上能够获得性能提升。此外,这种架构自然支持自适应计算和KV缓存共享等特性,暗示着它在实现更高效、更强大的语言模型方面具有很大的潜力。
原文链接:https://arxiv.org/abs/2502.05171