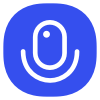
Sign up to save your podcasts
Or
Seventy3:借助NotebookLM的能力进行论文解读,专注人工智能、大模型、机器人算法方向,让大家跟着AI一起进步。
进群添加小助手微信:seventy3_podcast
备注:小宇宙
今天的主题是:LIMR: Less is More for RL ScalingSummary
This paper explores the efficiency of reinforcement learning (RL) data for enhancing large language models' reasoning abilities. It challenges the idea that more RL training data automatically leads to better performance. The authors introduce Learning Impact Measurement (LIM), a method to strategically select a small subset of highly impactful training samples. Their findings demonstrate that a carefully chosen fraction of data can achieve comparable or superior results compared to using the entire dataset. Furthermore, the research suggests that RL with smart data selection can outperform supervised fine-tuning for smaller models in data-scarce situations, highlighting the importance of data quality over quantity.
这篇论文探讨了强化学习(RL)数据在提升大型语言模型推理能力方面的效率。作者挑战了一个普遍的观点,即更多的RL训练数据一定能带来更好的性能。为了应对这一问题,作者提出了学习影响测量(LIM),一种通过战略性选择少量高影响力训练样本的方法。
研究结果表明,通过精心挑选一小部分数据,模型可以取得与使用整个数据集相当甚至更优的结果。此外,研究还表明,在数据稀缺的情况下,通过智能数据选择的RL能够在小型模型中超过监督微调的效果,强调了数据质量比数据数量更为重要。
原文链接:https://arxiv.org/abs/2502.11886
Seventy3:借助NotebookLM的能力进行论文解读,专注人工智能、大模型、机器人算法方向,让大家跟着AI一起进步。
进群添加小助手微信:seventy3_podcast
备注:小宇宙
今天的主题是:LIMR: Less is More for RL ScalingSummary
This paper explores the efficiency of reinforcement learning (RL) data for enhancing large language models' reasoning abilities. It challenges the idea that more RL training data automatically leads to better performance. The authors introduce Learning Impact Measurement (LIM), a method to strategically select a small subset of highly impactful training samples. Their findings demonstrate that a carefully chosen fraction of data can achieve comparable or superior results compared to using the entire dataset. Furthermore, the research suggests that RL with smart data selection can outperform supervised fine-tuning for smaller models in data-scarce situations, highlighting the importance of data quality over quantity.
这篇论文探讨了强化学习(RL)数据在提升大型语言模型推理能力方面的效率。作者挑战了一个普遍的观点,即更多的RL训练数据一定能带来更好的性能。为了应对这一问题,作者提出了学习影响测量(LIM),一种通过战略性选择少量高影响力训练样本的方法。
研究结果表明,通过精心挑选一小部分数据,模型可以取得与使用整个数据集相当甚至更优的结果。此外,研究还表明,在数据稀缺的情况下,通过智能数据选择的RL能够在小型模型中超过监督微调的效果,强调了数据质量比数据数量更为重要。
原文链接:https://arxiv.org/abs/2502.11886