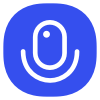
Sign up to save your podcasts
Or
Seventy3:借助NotebookLM的能力进行论文解读,专注人工智能、大模型、机器人算法方向,让大家跟着AI一起进步。
进群添加小助手微信:seventy3_podcast
备注:小宇宙
今天的主题是:MoBA: Mixture of Block Attention for Long-Context LLMsSummary
The provided technical report introduces Mixture of Block Attention (MoBA), a novel method to improve the efficiency of long-context large language models. MoBA applies the Mixture of Experts principle to the attention mechanism, allowing the model to selectively focus on relevant blocks of information instead of the entire context. This approach reduces the computational cost associated with traditional attention while maintaining strong performance on long-context tasks. Experiments demonstrate that MoBA achieves comparable scaling to full attention with significantly improved efficiency, and its flexibility allows for hybrid implementations and integration into existing models like Llama. Ultimately, MoBA offers a promising path towards more efficient and scalable processing of long sequences in large language models.
这份技术报告介绍了块注意力混合(MoBA),一种旨在提高长上下文大型语言模型效率的新方法。MoBA将**专家混合(Mixture of Experts)**原则应用于注意力机制,使模型能够选择性地关注相关的信息块,而非处理整个上下文。这种方法降低了传统注意力机制的计算成本,同时在长上下文任务中仍能保持强劲的表现。
实验结果表明,MoBA在效率上有显著提升,其扩展性与全注意力机制相当,并且提供了更多的灵活性,支持混合实现并能够集成到现有模型中,如Llama。最终,MoBA为在大型语言模型中更高效、可扩展地处理长序列提供了一个有前景的解决方案。
原文链接:https://arxiv.org/abs/2502.13189
Seventy3:借助NotebookLM的能力进行论文解读,专注人工智能、大模型、机器人算法方向,让大家跟着AI一起进步。
进群添加小助手微信:seventy3_podcast
备注:小宇宙
今天的主题是:MoBA: Mixture of Block Attention for Long-Context LLMsSummary
The provided technical report introduces Mixture of Block Attention (MoBA), a novel method to improve the efficiency of long-context large language models. MoBA applies the Mixture of Experts principle to the attention mechanism, allowing the model to selectively focus on relevant blocks of information instead of the entire context. This approach reduces the computational cost associated with traditional attention while maintaining strong performance on long-context tasks. Experiments demonstrate that MoBA achieves comparable scaling to full attention with significantly improved efficiency, and its flexibility allows for hybrid implementations and integration into existing models like Llama. Ultimately, MoBA offers a promising path towards more efficient and scalable processing of long sequences in large language models.
这份技术报告介绍了块注意力混合(MoBA),一种旨在提高长上下文大型语言模型效率的新方法。MoBA将**专家混合(Mixture of Experts)**原则应用于注意力机制,使模型能够选择性地关注相关的信息块,而非处理整个上下文。这种方法降低了传统注意力机制的计算成本,同时在长上下文任务中仍能保持强劲的表现。
实验结果表明,MoBA在效率上有显著提升,其扩展性与全注意力机制相当,并且提供了更多的灵活性,支持混合实现并能够集成到现有模型中,如Llama。最终,MoBA为在大型语言模型中更高效、可扩展地处理长序列提供了一个有前景的解决方案。
原文链接:https://arxiv.org/abs/2502.13189