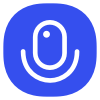
Sign up to save your podcasts
Or
Seventy3:借助NotebookLM的能力进行论文解读,专注人工智能、大模型、机器人算法方向,让大家跟着AI一起进步。
进群添加小助手微信:seventy3_podcast
备注:小宇宙
今天的主题是:Brain-to-Text Decoding: A Non-invasive Approach via TypingSummary
Researchers introduce Brain2Qwerty, a novel non-invasive brain-computer interface system that decodes typed sentences from the brain activity of healthy individuals using EEG and MEG. This new deep learning architecture translates brain signals recorded while participants typed memorized sentences on a QWERTY keyboard. The MEG-based decoding significantly outperformed EEG, achieving a notably lower character error rate and even correcting some typing mistakes. Analysis of the model's errors suggests it relies on motor processes linked to the keyboard layout, alongside higher-level cognitive functions, marking progress towards safer communication neuroprostheses.
研究人员提出了 Brain2Qwerty,这是一种新颖的非侵入式脑机接口系统,能够从健康个体的大脑活动中解码其输入的句子。该系统利用脑电图(EEG)和脑磁图(MEG)记录参与者在QWERTY键盘上输入记忆句子时的大脑信号,并通过深度学习架构进行翻译。实验显示,基于MEG的解码效果显著优于EEG,不仅字符错误率更低,甚至还纠正了一些输入错误。对模型错误的分析表明,其不仅依赖与键盘布局相关的运动过程,还涉及更高层次的认知功能,标志着向更安全的神经假体通信系统迈出了重要一步。
原文链接:https://arxiv.org/abs/2502.17480
Seventy3:借助NotebookLM的能力进行论文解读,专注人工智能、大模型、机器人算法方向,让大家跟着AI一起进步。
进群添加小助手微信:seventy3_podcast
备注:小宇宙
今天的主题是:Brain-to-Text Decoding: A Non-invasive Approach via TypingSummary
Researchers introduce Brain2Qwerty, a novel non-invasive brain-computer interface system that decodes typed sentences from the brain activity of healthy individuals using EEG and MEG. This new deep learning architecture translates brain signals recorded while participants typed memorized sentences on a QWERTY keyboard. The MEG-based decoding significantly outperformed EEG, achieving a notably lower character error rate and even correcting some typing mistakes. Analysis of the model's errors suggests it relies on motor processes linked to the keyboard layout, alongside higher-level cognitive functions, marking progress towards safer communication neuroprostheses.
研究人员提出了 Brain2Qwerty,这是一种新颖的非侵入式脑机接口系统,能够从健康个体的大脑活动中解码其输入的句子。该系统利用脑电图(EEG)和脑磁图(MEG)记录参与者在QWERTY键盘上输入记忆句子时的大脑信号,并通过深度学习架构进行翻译。实验显示,基于MEG的解码效果显著优于EEG,不仅字符错误率更低,甚至还纠正了一些输入错误。对模型错误的分析表明,其不仅依赖与键盘布局相关的运动过程,还涉及更高层次的认知功能,标志着向更安全的神经假体通信系统迈出了重要一步。
原文链接:https://arxiv.org/abs/2502.17480