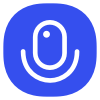
Sign up to save your podcasts
Or
Seventy3: 用NotebookLM将论文生成播客,让大家跟着AI一起进步。
今天的主题是:Diffusion Policies for Out-of-Distribution Generalization in Offline Reinforcement LearningThis briefing doc reviews the paper "Diffusion Policies for Out-of-Distribution Generalization in Offline Reinforcement Learning" by Ada, Oztop, and Ugur. The paper proposes a novel method, State Reconstruction for Diffusion Policies (SRDP), which improves upon existing diffusion-based ORL algorithms by tackling the challenge of out-of-distribution (OOD) state generalization.
Key Themes and Ideas:
Key Facts and Contributions:
Key Quotes:
Future Directions:
The paper suggests evaluating SRDP on more challenging ORL tasks specifically designed for OOD generalization. Further research could explore the application of SRDP in real-world domains and investigate its potential for improving safety and reliability in areas like autonomous driving and robotics.
Overall:
This paper presents a significant advancement in addressing OOD state generalization within the context of ORL. SRDP demonstrates promising results on benchmark tasks and provides a valuable foundation for future research in this critical area.
原文链接:https://arxiv.org/abs/2307.04726
Seventy3: 用NotebookLM将论文生成播客,让大家跟着AI一起进步。
今天的主题是:Diffusion Policies for Out-of-Distribution Generalization in Offline Reinforcement LearningThis briefing doc reviews the paper "Diffusion Policies for Out-of-Distribution Generalization in Offline Reinforcement Learning" by Ada, Oztop, and Ugur. The paper proposes a novel method, State Reconstruction for Diffusion Policies (SRDP), which improves upon existing diffusion-based ORL algorithms by tackling the challenge of out-of-distribution (OOD) state generalization.
Key Themes and Ideas:
Key Facts and Contributions:
Key Quotes:
Future Directions:
The paper suggests evaluating SRDP on more challenging ORL tasks specifically designed for OOD generalization. Further research could explore the application of SRDP in real-world domains and investigate its potential for improving safety and reliability in areas like autonomous driving and robotics.
Overall:
This paper presents a significant advancement in addressing OOD state generalization within the context of ORL. SRDP demonstrates promising results on benchmark tasks and provides a valuable foundation for future research in this critical area.
原文链接:https://arxiv.org/abs/2307.04726