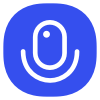
Sign up to save your podcasts
Or
Seventy3:借助NotebookLM的能力进行论文解读,专注人工智能、大模型、机器人算法方向,让大家跟着AI一起进步。
进群添加小助手微信:seventy3_podcast
备注:小宇宙
今天的主题是:On the Emergence of Thinking in LLMs I: Searching for the Right IntuitionSummary
The provided research explores how large language models (LLMs) can be transformed into more capable reasoning agents, termed large reasoning models (LRMs), by enabling a "thinking" process during inference. It introduces Reinforcement Learning via Self-Play (RLSP), a post-training framework that encourages guided search in LLMs through supervised fine-tuning on reasoning demonstrations, an exploration reward for diverse behavior, and reinforcement learning with an outcome verifier. Empirical results in mathematical problem-solving show that RLSP enhances reasoning abilities and fosters emergent behaviors like backtracking and self-correction across different model architectures and sizes. The work posits that RLSP's approach to incentivize the generation of novel reasoning trajectories through self-play contributes to the improved computational power and problem-solving capabilities of LLMs.
该研究探讨了如何将大型语言模型(LLMs)转化为更具推理能力的智能体,即“大型推理模型”(LRMs),通过在推理过程中引入“思考”机制实现能力提升。作者提出了一种名为“自对弈强化学习”(RLSP)的后训练框架,旨在引导LLMs进行有目标的搜索。该框架结合了基于推理示例的有监督微调、用于鼓励多样化行为的探索奖励,以及配备结果验证器的强化学习。在数学问题求解任务中的实验证明,RLSP显著提升了模型的推理能力,并促发了如回溯、自我纠错等新兴行为,在不同模型架构和规模中均表现出良好适应性。该研究认为,RLSP通过自对弈激励生成新颖的推理路径,有效提升了LLMs的计算能力与问题解决能力。
原文链接:https://arxiv.org/abs/2502.06773
Seventy3:借助NotebookLM的能力进行论文解读,专注人工智能、大模型、机器人算法方向,让大家跟着AI一起进步。
进群添加小助手微信:seventy3_podcast
备注:小宇宙
今天的主题是:On the Emergence of Thinking in LLMs I: Searching for the Right IntuitionSummary
The provided research explores how large language models (LLMs) can be transformed into more capable reasoning agents, termed large reasoning models (LRMs), by enabling a "thinking" process during inference. It introduces Reinforcement Learning via Self-Play (RLSP), a post-training framework that encourages guided search in LLMs through supervised fine-tuning on reasoning demonstrations, an exploration reward for diverse behavior, and reinforcement learning with an outcome verifier. Empirical results in mathematical problem-solving show that RLSP enhances reasoning abilities and fosters emergent behaviors like backtracking and self-correction across different model architectures and sizes. The work posits that RLSP's approach to incentivize the generation of novel reasoning trajectories through self-play contributes to the improved computational power and problem-solving capabilities of LLMs.
该研究探讨了如何将大型语言模型(LLMs)转化为更具推理能力的智能体,即“大型推理模型”(LRMs),通过在推理过程中引入“思考”机制实现能力提升。作者提出了一种名为“自对弈强化学习”(RLSP)的后训练框架,旨在引导LLMs进行有目标的搜索。该框架结合了基于推理示例的有监督微调、用于鼓励多样化行为的探索奖励,以及配备结果验证器的强化学习。在数学问题求解任务中的实验证明,RLSP显著提升了模型的推理能力,并促发了如回溯、自我纠错等新兴行为,在不同模型架构和规模中均表现出良好适应性。该研究认为,RLSP通过自对弈激励生成新颖的推理路径,有效提升了LLMs的计算能力与问题解决能力。
原文链接:https://arxiv.org/abs/2502.06773