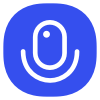
Sign up to save your podcasts
Or
Seventy3: 用NotebookLM将论文生成播客,让大家跟着AI一起进步。
今天的主题是:Diffusion Policies as an Expressive Policy Class for Offline Reinforcement LearningSource: Wang, Z., Hunt, J.J., & Zhou, M. (2023). Diffusion Policies as an Expressive Policy Class for Offline Reinforcement Learning. arXiv preprint arXiv:2208.06193v3.
Main Theme: This paper proposes Diffusion Q-learning (Diffusion-QL), a novel offline reinforcement learning (RL) algorithm that utilizes diffusion models for precise policy regularization and leverages Q-learning guidance to achieve state-of-the-art performance on benchmark tasks.
Most Important Ideas/Facts:
Overall, Diffusion-QL presents a significant advancement in offline RL by leveraging the power of diffusion models for policy regularization. The algorithm effectively addresses the limitations of existing methods and demonstrates superior performance on challenging benchmark tasks, offering promising avenues for future research in the field.
原文链接:https://arxiv.org/abs/2208.06193
Seventy3: 用NotebookLM将论文生成播客,让大家跟着AI一起进步。
今天的主题是:Diffusion Policies as an Expressive Policy Class for Offline Reinforcement LearningSource: Wang, Z., Hunt, J.J., & Zhou, M. (2023). Diffusion Policies as an Expressive Policy Class for Offline Reinforcement Learning. arXiv preprint arXiv:2208.06193v3.
Main Theme: This paper proposes Diffusion Q-learning (Diffusion-QL), a novel offline reinforcement learning (RL) algorithm that utilizes diffusion models for precise policy regularization and leverages Q-learning guidance to achieve state-of-the-art performance on benchmark tasks.
Most Important Ideas/Facts:
Overall, Diffusion-QL presents a significant advancement in offline RL by leveraging the power of diffusion models for policy regularization. The algorithm effectively addresses the limitations of existing methods and demonstrates superior performance on challenging benchmark tasks, offering promising avenues for future research in the field.
原文链接:https://arxiv.org/abs/2208.06193