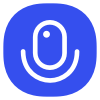
Sign up to save your podcasts
Or
Seventy3: 用NotebookLM将论文生成播客,让大家跟着AI一起进步。
今天的主题是:Diffusion Models are Evolutionary AlgorithmsSummary
This research paper proposes a novel approach to evolutionary algorithms called Diffusion Evolution, which draws a parallel between the process of biological evolution and the mathematical framework of diffusion models in machine learning. The authors demonstrate that diffusion models can be interpreted as performing evolutionary algorithms, inherently encompassing selection, mutation, and reproductive isolation. By utilizing the denoising process of diffusion models, the Diffusion Evolution method efficiently identifies multiple optimal solutions in complex parameter spaces, outperforming traditional evolutionary algorithms. Furthermore, the paper introduces Latent Space Diffusion Evolution, which leverages latent space diffusion to find solutions for evolutionary tasks in high-dimensional parameter spaces while significantly reducing computational steps. This new understanding of the connection between diffusion and evolution not only bridges two distinct fields but also opens new avenues for mutual enhancement and raises questions about open-ended evolution and the potential utilization of non-Gaussian or discrete diffusion models in the context of Diffusion Evolution.
原文链接:https://arxiv.org/abs/2410.02543v2
Seventy3: 用NotebookLM将论文生成播客,让大家跟着AI一起进步。
今天的主题是:Diffusion Models are Evolutionary AlgorithmsSummary
This research paper proposes a novel approach to evolutionary algorithms called Diffusion Evolution, which draws a parallel between the process of biological evolution and the mathematical framework of diffusion models in machine learning. The authors demonstrate that diffusion models can be interpreted as performing evolutionary algorithms, inherently encompassing selection, mutation, and reproductive isolation. By utilizing the denoising process of diffusion models, the Diffusion Evolution method efficiently identifies multiple optimal solutions in complex parameter spaces, outperforming traditional evolutionary algorithms. Furthermore, the paper introduces Latent Space Diffusion Evolution, which leverages latent space diffusion to find solutions for evolutionary tasks in high-dimensional parameter spaces while significantly reducing computational steps. This new understanding of the connection between diffusion and evolution not only bridges two distinct fields but also opens new avenues for mutual enhancement and raises questions about open-ended evolution and the potential utilization of non-Gaussian or discrete diffusion models in the context of Diffusion Evolution.
原文链接:https://arxiv.org/abs/2410.02543v2