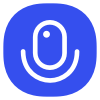
Sign up to save your podcasts
Or
Seventy3: 用NotebookLM将论文生成播客,让大家跟着AI一起进步。
今天的主题是:Rethinking Softmax: Self-Attention with Polynomial ActivationsSummary
This research paper examines the effectiveness of the softmax activation function in transformer architectures, commonly used for attention mechanisms. The authors argue that softmax's success stems not solely from its ability to produce a probability distribution for attention allocation but also from its implicit regularization of the Frobenius norm of the attention matrix. They present a theoretical framework for deriving polynomial activations that achieve similar regularization effects, even though they may violate the typical properties of softmax attention. The paper demonstrates that these alternative activations can perform comparably or better than softmax across various vision and NLP tasks, suggesting new possibilities for attention mechanisms beyond the traditional softmax approach.
原文链接:https://arxiv.org/abs/2410.18613
Seventy3: 用NotebookLM将论文生成播客,让大家跟着AI一起进步。
今天的主题是:Rethinking Softmax: Self-Attention with Polynomial ActivationsSummary
This research paper examines the effectiveness of the softmax activation function in transformer architectures, commonly used for attention mechanisms. The authors argue that softmax's success stems not solely from its ability to produce a probability distribution for attention allocation but also from its implicit regularization of the Frobenius norm of the attention matrix. They present a theoretical framework for deriving polynomial activations that achieve similar regularization effects, even though they may violate the typical properties of softmax attention. The paper demonstrates that these alternative activations can perform comparably or better than softmax across various vision and NLP tasks, suggesting new possibilities for attention mechanisms beyond the traditional softmax approach.
原文链接:https://arxiv.org/abs/2410.18613