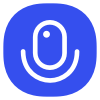
Sign up to save your podcasts
Or
Seventy3: 用NotebookLM将论文生成播客,让大家跟着AI一起进步。
今天的主题是:Scaling Proprioceptive-Visual Learning with Heterogeneous Pre-trained TransformersSummary
This research paper proposes a new architecture called Heterogeneous Pre-trained Transformers (HPT) to address the challenges of training generalist robotic models. HPT leverages a shared "trunk" transformer network to learn a task-agnostic and embodiment-agnostic representation from diverse robotic datasets, including real-world robots, simulations, and human videos. The paper demonstrates that HPT scales effectively with increasing dataset size, model size, and training compute. Importantly, HPT's learned representations can be transferred to new embodiments, tasks, and environments, improving performance in both simulation and real-world settings.
原文链接:https://arxiv.org/abs/2409.20537
英文解读:https://news.mit.edu/2024/training-general-purpose-robots-faster-better-1028
Seventy3: 用NotebookLM将论文生成播客,让大家跟着AI一起进步。
今天的主题是:Scaling Proprioceptive-Visual Learning with Heterogeneous Pre-trained TransformersSummary
This research paper proposes a new architecture called Heterogeneous Pre-trained Transformers (HPT) to address the challenges of training generalist robotic models. HPT leverages a shared "trunk" transformer network to learn a task-agnostic and embodiment-agnostic representation from diverse robotic datasets, including real-world robots, simulations, and human videos. The paper demonstrates that HPT scales effectively with increasing dataset size, model size, and training compute. Importantly, HPT's learned representations can be transferred to new embodiments, tasks, and environments, improving performance in both simulation and real-world settings.
原文链接:https://arxiv.org/abs/2409.20537
英文解读:https://news.mit.edu/2024/training-general-purpose-robots-faster-better-1028