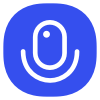
Sign up to save your podcasts
Or
Seventy3: 用NotebookLM将论文生成播客,让大家跟着AI一起进步。
今天的主题是:Precise and Dexterous Robotic Manipulation via Human-in-the-Loop Reinforcement LearningSummary
The research paper "Precise and Dexterous Robotic Manipulation via Human-in-the-Loop Reinforcement Learning" investigates the effectiveness of human-in-the-loop reinforcement learning (HIL-SERL) for training robots to perform complex manipulation tasks. The researchers present a system that combines human demonstrations and corrections with sample-efficient reinforcement learning algorithms to train robots on a diverse set of dexterous manipulation tasks, including dynamic manipulation, precision assembly, and dual-arm coordination. Their findings show that HIL-SERL significantly outperforms imitation learning baselines and prior RL approaches, achieving near-perfect success rates and fast cycle times within just 1 to 2.5 hours of training. The paper also explores the reliability and learned behaviors of the policies, demonstrating their ability to adapt dynamically to variations and handle external disturbances. The research highlights the potential of HIL-SERL as a general framework for acquiring a wide range of manipulation skills with high performance and adaptability, paving the way for the use of reinforcement learning in solving real-world robotic manipulation problems.
原文链接:https://hil-serl.github.io
解读:强化学习训练一两个小时,100%自主完成任务:机器人ChatGPT时刻真来了?
Seventy3: 用NotebookLM将论文生成播客,让大家跟着AI一起进步。
今天的主题是:Precise and Dexterous Robotic Manipulation via Human-in-the-Loop Reinforcement LearningSummary
The research paper "Precise and Dexterous Robotic Manipulation via Human-in-the-Loop Reinforcement Learning" investigates the effectiveness of human-in-the-loop reinforcement learning (HIL-SERL) for training robots to perform complex manipulation tasks. The researchers present a system that combines human demonstrations and corrections with sample-efficient reinforcement learning algorithms to train robots on a diverse set of dexterous manipulation tasks, including dynamic manipulation, precision assembly, and dual-arm coordination. Their findings show that HIL-SERL significantly outperforms imitation learning baselines and prior RL approaches, achieving near-perfect success rates and fast cycle times within just 1 to 2.5 hours of training. The paper also explores the reliability and learned behaviors of the policies, demonstrating their ability to adapt dynamically to variations and handle external disturbances. The research highlights the potential of HIL-SERL as a general framework for acquiring a wide range of manipulation skills with high performance and adaptability, paving the way for the use of reinforcement learning in solving real-world robotic manipulation problems.
原文链接:https://hil-serl.github.io
解读:强化学习训练一两个小时,100%自主完成任务:机器人ChatGPT时刻真来了?