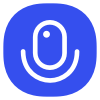
Sign up to save your podcasts
Or
Seventy3: 用NotebookLM将论文生成播客,让大家跟着AI一起进步。
今天的主题是:The Geometry of Concepts: Sparse Autoencoder Feature StructureSummary
This research paper investigates the structure of the "concept universe" within large language models (LLMs), specifically focusing on sparse autoencoders (SAEs). The authors examine the organization of SAE features at three distinct scales. At the atomic scale, they discover "crystals" reflecting semantic relations between concepts, similar to the well-known "king:man::queen:woman" analogy. At the brain scale, they demonstrate that functionally related SAE features cluster together spatially, forming "lobes" reminiscent of functional areas in the human brain. Finally, at the galaxy scale, the authors analyze the overall shape and clustering of the SAE feature space, finding a power law distribution of eigenvalues and revealing a surprising degree of clustering.
原文链接:https://arxiv.org/abs/2410.19750
Seventy3: 用NotebookLM将论文生成播客,让大家跟着AI一起进步。
今天的主题是:The Geometry of Concepts: Sparse Autoencoder Feature StructureSummary
This research paper investigates the structure of the "concept universe" within large language models (LLMs), specifically focusing on sparse autoencoders (SAEs). The authors examine the organization of SAE features at three distinct scales. At the atomic scale, they discover "crystals" reflecting semantic relations between concepts, similar to the well-known "king:man::queen:woman" analogy. At the brain scale, they demonstrate that functionally related SAE features cluster together spatially, forming "lobes" reminiscent of functional areas in the human brain. Finally, at the galaxy scale, the authors analyze the overall shape and clustering of the SAE feature space, finding a power law distribution of eigenvalues and revealing a surprising degree of clustering.
原文链接:https://arxiv.org/abs/2410.19750