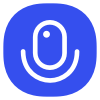
Sign up to save your podcasts
Or
Seventy3: 用NotebookLM将论文生成播客,让大家跟着AI一起进步。
今天的主题是:AFlow: Automating Agentic Workflow GenerationSummary
This research paper presents AFLOW, a novel framework for automated workflow optimization for large language models (LLMs). It tackles the challenge of manually designing and refining agentic workflows, which are structured sequences of LLM invocations, by using Monte Carlo Tree Search (MCTS) to explore the vast search space of possible workflows. AFLOW represents these workflows as code-represented nodes connected by edges, allowing it to efficiently navigate and refine workflows through iterative modification, experience-based learning, and execution feedback. The paper showcases AFLOW's effectiveness across six benchmark datasets, demonstrating its ability to outperform both manually designed methods and existing automated approaches. It also highlights AFLOW's ability to enable smaller models to achieve better performance than larger models at significantly lower costs.
原文链接:https://arxiv.org/abs/2410.10762
Seventy3: 用NotebookLM将论文生成播客,让大家跟着AI一起进步。
今天的主题是:AFlow: Automating Agentic Workflow GenerationSummary
This research paper presents AFLOW, a novel framework for automated workflow optimization for large language models (LLMs). It tackles the challenge of manually designing and refining agentic workflows, which are structured sequences of LLM invocations, by using Monte Carlo Tree Search (MCTS) to explore the vast search space of possible workflows. AFLOW represents these workflows as code-represented nodes connected by edges, allowing it to efficiently navigate and refine workflows through iterative modification, experience-based learning, and execution feedback. The paper showcases AFLOW's effectiveness across six benchmark datasets, demonstrating its ability to outperform both manually designed methods and existing automated approaches. It also highlights AFLOW's ability to enable smaller models to achieve better performance than larger models at significantly lower costs.
原文链接:https://arxiv.org/abs/2410.10762