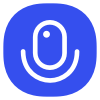
Sign up to save your podcasts
Or
Seventy3: 用NotebookLM将论文生成播客,让大家跟着AI一起进步。
今天的主题是:Multi-Agent Coordination via Multi-Level CommunicationSummary
This research paper introduces a novel multi-agent communication scheme called Sequential Communication (SeqComm) that aims to improve coordination in cooperative multi-agent reinforcement learning (MARL) tasks. SeqComm tackles the coordination problem by treating agents asynchronously, allowing them to make decisions sequentially based on the actions of higher-level agents. The paper presents a theoretical analysis of SeqComm's performance, demonstrating that the learned policies improve monotonically and converge. Furthermore, empirical results on the StarCraft Multi-Agent Challenge v2 (SMACv2) benchmark show that SeqComm outperforms existing methods, highlighting the effectiveness of its approach to promoting explicit coordination among agents.
原文链接:https://arxiv.org/abs/2209.12713
Seventy3: 用NotebookLM将论文生成播客,让大家跟着AI一起进步。
今天的主题是:Multi-Agent Coordination via Multi-Level CommunicationSummary
This research paper introduces a novel multi-agent communication scheme called Sequential Communication (SeqComm) that aims to improve coordination in cooperative multi-agent reinforcement learning (MARL) tasks. SeqComm tackles the coordination problem by treating agents asynchronously, allowing them to make decisions sequentially based on the actions of higher-level agents. The paper presents a theoretical analysis of SeqComm's performance, demonstrating that the learned policies improve monotonically and converge. Furthermore, empirical results on the StarCraft Multi-Agent Challenge v2 (SMACv2) benchmark show that SeqComm outperforms existing methods, highlighting the effectiveness of its approach to promoting explicit coordination among agents.
原文链接:https://arxiv.org/abs/2209.12713