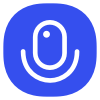
Sign up to save your podcasts
Or
Seventy3: 用NotebookLM将论文生成播客,让大家跟着AI一起进步。
今天的主题是:Will we run out of data? Limits of LLM scaling based on human-generated dataSummary
This research paper investigates whether the limited availability of public human text data could constrain the continued scaling of large language models (LLMs). The authors use statistical models to predict when the total available stock of text data will be exhausted based on current LLM development trends, concluding that this could happen as early as 2026. The paper then examines several potential strategies to circumvent this data bottleneck, including using models to generate synthetic data, transfer learning from data-rich domains, and the use of non-public data. Ultimately, the authors conclude that while a data bottleneck is imminent, progress in LLM development can continue through the adoption of these alternative data sources and techniques.
原文链接:https://arxiv.org/abs/2211.04325
Seventy3: 用NotebookLM将论文生成播客,让大家跟着AI一起进步。
今天的主题是:Will we run out of data? Limits of LLM scaling based on human-generated dataSummary
This research paper investigates whether the limited availability of public human text data could constrain the continued scaling of large language models (LLMs). The authors use statistical models to predict when the total available stock of text data will be exhausted based on current LLM development trends, concluding that this could happen as early as 2026. The paper then examines several potential strategies to circumvent this data bottleneck, including using models to generate synthetic data, transfer learning from data-rich domains, and the use of non-public data. Ultimately, the authors conclude that while a data bottleneck is imminent, progress in LLM development can continue through the adoption of these alternative data sources and techniques.
原文链接:https://arxiv.org/abs/2211.04325