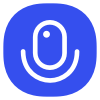
Sign up to save your podcasts
Or
Seventy3: 用NotebookLM将论文生成播客,让大家跟着AI一起进步。
今天的主题是:LoRA vs Full Fine-tuning: An Illusion of EquivalenceSummary
This research paper investigates the differences between two popular methods for fine-tuning large language models: full fine-tuning and Low-Rank Adaptation (LoRA). While both approaches can achieve comparable performance on downstream tasks, the authors show that these methods learn fundamentally different solutions. They analyze the spectral properties of weight matrices to identify "intruder dimensions" - singular vectors that appear in LoRA models but not in fully fine-tuned models. These intruder dimensions contribute to a phenomenon where LoRA models exhibit less robust generalization than full fine-tuning, especially when trained on multiple tasks sequentially. The authors further explore how the design choices in LoRA, such as rank and the scaling factor α, affect the emergence of intruder dimensions and the overall performance of the models. They conclude that although LoRA can achieve comparable performance to full fine-tuning on specific tasks, it might not be the optimal choice for scenarios requiring robust generalization and continual learning.
原文链接:https://arxiv.org/abs/2410.21228
Seventy3: 用NotebookLM将论文生成播客,让大家跟着AI一起进步。
今天的主题是:LoRA vs Full Fine-tuning: An Illusion of EquivalenceSummary
This research paper investigates the differences between two popular methods for fine-tuning large language models: full fine-tuning and Low-Rank Adaptation (LoRA). While both approaches can achieve comparable performance on downstream tasks, the authors show that these methods learn fundamentally different solutions. They analyze the spectral properties of weight matrices to identify "intruder dimensions" - singular vectors that appear in LoRA models but not in fully fine-tuned models. These intruder dimensions contribute to a phenomenon where LoRA models exhibit less robust generalization than full fine-tuning, especially when trained on multiple tasks sequentially. The authors further explore how the design choices in LoRA, such as rank and the scaling factor α, affect the emergence of intruder dimensions and the overall performance of the models. They conclude that although LoRA can achieve comparable performance to full fine-tuning on specific tasks, it might not be the optimal choice for scenarios requiring robust generalization and continual learning.
原文链接:https://arxiv.org/abs/2410.21228