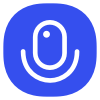
Sign up to save your podcasts
Or
Seventy3: 用NotebookLM将论文生成播客,让大家跟着AI一起进步。
今天的主题是:AM-RADIO: Agglomerative Vision Foundation Model -- Reduce All Domains Into OneSummary
This paper proposes a new approach to training vision foundation models (VFMs) called AM-RADIO, which agglomerates the unique strengths of multiple pretrained models like CLIP, DINOv2, and SAM into a single model. The framework uses multi-teacher distillation to achieve this, and the resulting models outperform individual teacher models on various downstream tasks like classification, segmentation, and vision-language modeling. Notably, a new architecture called E-RADIO is introduced, which is significantly more efficient than traditional ViTs, allowing for faster inference and comparable performance. The paper thoroughly analyzes the effectiveness of the AM-RADIO approach, providing comprehensive results and insights into the distillation process.
原文链接:https://arxiv.org/abs/2312.06709
Seventy3: 用NotebookLM将论文生成播客,让大家跟着AI一起进步。
今天的主题是:AM-RADIO: Agglomerative Vision Foundation Model -- Reduce All Domains Into OneSummary
This paper proposes a new approach to training vision foundation models (VFMs) called AM-RADIO, which agglomerates the unique strengths of multiple pretrained models like CLIP, DINOv2, and SAM into a single model. The framework uses multi-teacher distillation to achieve this, and the resulting models outperform individual teacher models on various downstream tasks like classification, segmentation, and vision-language modeling. Notably, a new architecture called E-RADIO is introduced, which is significantly more efficient than traditional ViTs, allowing for faster inference and comparable performance. The paper thoroughly analyzes the effectiveness of the AM-RADIO approach, providing comprehensive results and insights into the distillation process.
原文链接:https://arxiv.org/abs/2312.06709