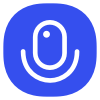
Sign up to save your podcasts
Or
Seventy3: 用NotebookLM将论文生成播客,让大家跟着AI一起进步。
今天的主题是:SymDPO: Boosting In-Context Learning of Large Multimodal Models with Symbol Demonstration Direct Preference OptimizationSummary
This research introduces SymDPO, a novel method to improve the in-context learning capabilities of Large Multimodal Models (LMMs). Current LMMs often prioritize textual information over visual context in demonstrations, leading to inaccurate results. SymDPO addresses this "visual context overlook" by replacing text answers with symbols, forcing the model to rely on both visual and symbolic cues for correct responses. Experiments across various benchmarks demonstrate that SymDPO significantly enhances LMM performance compared to existing methods like General DPO, Video DPO, and MIA-DPO. The improved performance highlights SymDPO's success in fostering a more balanced understanding of multimodal information within in-context learning scenarios.
原文链接:https://arxiv.org/abs/2411.11909
Seventy3: 用NotebookLM将论文生成播客,让大家跟着AI一起进步。
今天的主题是:SymDPO: Boosting In-Context Learning of Large Multimodal Models with Symbol Demonstration Direct Preference OptimizationSummary
This research introduces SymDPO, a novel method to improve the in-context learning capabilities of Large Multimodal Models (LMMs). Current LMMs often prioritize textual information over visual context in demonstrations, leading to inaccurate results. SymDPO addresses this "visual context overlook" by replacing text answers with symbols, forcing the model to rely on both visual and symbolic cues for correct responses. Experiments across various benchmarks demonstrate that SymDPO significantly enhances LMM performance compared to existing methods like General DPO, Video DPO, and MIA-DPO. The improved performance highlights SymDPO's success in fostering a more balanced understanding of multimodal information within in-context learning scenarios.
原文链接:https://arxiv.org/abs/2411.11909