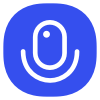
Sign up to save your podcasts
Or
Seventy3: 用NotebookLM将论文生成播客,让大家跟着AI一起进步。
今天的主题是:Procedural Knowledge in Pretraining Drives Reasoning in Large Language ModelsSummary
This research investigates how large language models (LLMs) learn to reason, contrasting their strategies for reasoning tasks with those used for factual recall. The study analyzes the influence of pretraining data on model outputs for mathematical reasoning and factual questions, revealing that LLMs utilize procedural knowledge from the pretraining data rather than simple retrieval for reasoning. The findings indicate that LLMs rely less on individual documents for reasoning and show stronger correlations between document influence across similar reasoning problems. Importantly, the presence of code in the pretraining data is highlighted as a significant factor influencing the LLMs' reasoning capabilities. The study's results offer insights into improving LLM reasoning by focusing pretraining data selection on high-quality procedural knowledge examples. Limitations are acknowledged, particularly concerning the inability to analyze the entire pretraining dataset.
原文链接:https://arxiv.org/abs/2411.12580
解读链接:https://www.jiqizhixin.com/articles/2024-11-22-2
Seventy3: 用NotebookLM将论文生成播客,让大家跟着AI一起进步。
今天的主题是:Procedural Knowledge in Pretraining Drives Reasoning in Large Language ModelsSummary
This research investigates how large language models (LLMs) learn to reason, contrasting their strategies for reasoning tasks with those used for factual recall. The study analyzes the influence of pretraining data on model outputs for mathematical reasoning and factual questions, revealing that LLMs utilize procedural knowledge from the pretraining data rather than simple retrieval for reasoning. The findings indicate that LLMs rely less on individual documents for reasoning and show stronger correlations between document influence across similar reasoning problems. Importantly, the presence of code in the pretraining data is highlighted as a significant factor influencing the LLMs' reasoning capabilities. The study's results offer insights into improving LLM reasoning by focusing pretraining data selection on high-quality procedural knowledge examples. Limitations are acknowledged, particularly concerning the inability to analyze the entire pretraining dataset.
原文链接:https://arxiv.org/abs/2411.12580
解读链接:https://www.jiqizhixin.com/articles/2024-11-22-2