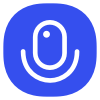
Sign up to save your podcasts
Or
Seventy3: 用NotebookLM将论文生成播客,让大家跟着AI一起进步。
今天的主题是:Deep Reinforcement Learning Without Experience Replay, Target Networks, or Batch UpdatesSummary
This research paper introduces stream-x algorithms, a novel class of deep reinforcement learning algorithms designed for streaming data. Unlike traditional deep RL methods that rely on computationally expensive batch updates and experience replay, stream-x processes individual samples in real time. The authors address the "stream barrier"—the instability and learning failures common in streaming deep RL—through several techniques including a novel optimizer, data scaling, and sparse initialization. Experiments across various benchmark environments demonstrate that stream-x algorithms achieve comparable sample efficiency and performance to batch methods, sometimes surpassing them. The study challenges the prevailing assumption that streaming deep RL is inherently sample-inefficient.
原文链接:https://openreview.net/forum?id=yqQJGTDGXN
Seventy3: 用NotebookLM将论文生成播客,让大家跟着AI一起进步。
今天的主题是:Deep Reinforcement Learning Without Experience Replay, Target Networks, or Batch UpdatesSummary
This research paper introduces stream-x algorithms, a novel class of deep reinforcement learning algorithms designed for streaming data. Unlike traditional deep RL methods that rely on computationally expensive batch updates and experience replay, stream-x processes individual samples in real time. The authors address the "stream barrier"—the instability and learning failures common in streaming deep RL—through several techniques including a novel optimizer, data scaling, and sparse initialization. Experiments across various benchmark environments demonstrate that stream-x algorithms achieve comparable sample efficiency and performance to batch methods, sometimes surpassing them. The study challenges the prevailing assumption that streaming deep RL is inherently sample-inefficient.
原文链接:https://openreview.net/forum?id=yqQJGTDGXN