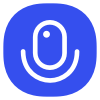
Sign up to save your podcasts
Or
Seventy3: 用NotebookLM将论文生成播客,让大家跟着AI一起进步。
今天的主题是:Beyond Examples: High-level Automated Reasoning Paradigm in In-Context Learning via MCTSSummary
This research paper introduces HiAR-ICL, a novel framework for improving in-context learning (ICL) in large language models (LLMs), particularly for complex mathematical reasoning. Instead of relying solely on example demonstrations, HiAR-ICL uses Monte Carlo Tree Search (MCTS) to automatically generate and select higher-level reasoning patterns, effectively "teaching the LLM to think" rather than just mimicking examples. The approach uses five atomic reasoning actions as building blocks for these patterns, and a cognitive complexity framework to match problems with appropriate patterns. Experimental results show HiAR-ICL achieves state-of-the-art accuracy on several benchmarks, surpassing even some closed-source LLMs, especially when used with smaller, open-source models.
原文链接:https://arxiv.org/abs/2411.18478
Seventy3: 用NotebookLM将论文生成播客,让大家跟着AI一起进步。
今天的主题是:Beyond Examples: High-level Automated Reasoning Paradigm in In-Context Learning via MCTSSummary
This research paper introduces HiAR-ICL, a novel framework for improving in-context learning (ICL) in large language models (LLMs), particularly for complex mathematical reasoning. Instead of relying solely on example demonstrations, HiAR-ICL uses Monte Carlo Tree Search (MCTS) to automatically generate and select higher-level reasoning patterns, effectively "teaching the LLM to think" rather than just mimicking examples. The approach uses five atomic reasoning actions as building blocks for these patterns, and a cognitive complexity framework to match problems with appropriate patterns. Experimental results show HiAR-ICL achieves state-of-the-art accuracy on several benchmarks, surpassing even some closed-source LLMs, especially when used with smaller, open-source models.
原文链接:https://arxiv.org/abs/2411.18478