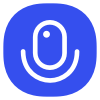
Sign up to save your podcasts
Or
Seventy3: 用NotebookLM将论文生成播客,让大家跟着AI一起进步。
今天的主题是:Training Large Language Models to Reason in a Continuous Latent SpaceSummary
This research paper introduces Coconut, a novel method for enhancing Large Language Model (LLM) reasoning capabilities. Instead of relying solely on language-based chain-of-thought (CoT) reasoning, Coconut utilizes the LLM's hidden state ("continuous thought") as input, enabling reasoning in an unrestricted latent space. Experiments on various reasoning tasks demonstrate that Coconut outperforms traditional CoT methods, especially in tasks requiring significant planning and backtracking. The study analyzes the emergent breadth-first search-like reasoning pattern in Coconut and explores the advantages of latent reasoning over language-based approaches. The findings suggest promising avenues for future research in improving LLM reasoning.
这篇研究论文介绍了一种名为 Coconut 的新方法,用于增强大型语言模型(LLM)的推理能力。与仅依赖基于语言的链式思维(CoT)推理不同,Coconut 利用 LLM 的隐藏状态(“连续思维”)作为输入,从而实现了在无限制的潜在空间中进行推理。在多种推理任务上的实验表明,Coconut 优于传统的 CoT 方法,特别是在需要大量规划和回溯的任务中。研究分析了 Coconut 中呈现的类似广度优先搜索的推理模式,并探讨了潜在推理相较于基于语言方法的优势。研究结果为未来在改进 LLM 推理方面提供了有前景的研究方向。
原文链接:https://arxiv.org/abs/2412.06769
Seventy3: 用NotebookLM将论文生成播客,让大家跟着AI一起进步。
今天的主题是:Training Large Language Models to Reason in a Continuous Latent SpaceSummary
This research paper introduces Coconut, a novel method for enhancing Large Language Model (LLM) reasoning capabilities. Instead of relying solely on language-based chain-of-thought (CoT) reasoning, Coconut utilizes the LLM's hidden state ("continuous thought") as input, enabling reasoning in an unrestricted latent space. Experiments on various reasoning tasks demonstrate that Coconut outperforms traditional CoT methods, especially in tasks requiring significant planning and backtracking. The study analyzes the emergent breadth-first search-like reasoning pattern in Coconut and explores the advantages of latent reasoning over language-based approaches. The findings suggest promising avenues for future research in improving LLM reasoning.
这篇研究论文介绍了一种名为 Coconut 的新方法,用于增强大型语言模型(LLM)的推理能力。与仅依赖基于语言的链式思维(CoT)推理不同,Coconut 利用 LLM 的隐藏状态(“连续思维”)作为输入,从而实现了在无限制的潜在空间中进行推理。在多种推理任务上的实验表明,Coconut 优于传统的 CoT 方法,特别是在需要大量规划和回溯的任务中。研究分析了 Coconut 中呈现的类似广度优先搜索的推理模式,并探讨了潜在推理相较于基于语言方法的优势。研究结果为未来在改进 LLM 推理方面提供了有前景的研究方向。
原文链接:https://arxiv.org/abs/2412.06769