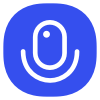
Sign up to save your podcasts
Or
Seventy3: 用NotebookLM将论文生成播客,让大家跟着AI一起进步。
今天的主题是:Segment Any Text: A Universal Approach for Robust, Efficient and Adaptable Sentence SegmentationSummary
This research paper introduces Segment Any Text (SAT), a novel sentence segmentation model that surpasses existing methods. SAT achieves robustness by reducing reliance on punctuation during training, demonstrates adaptability through parameter-efficient fine-tuning across diverse domains (e.g., lyrics, legal texts), and boasts high efficiency, outperforming even strong large language models (LLMs). The authors detail SAT's architecture, training process, and extensive evaluation across multiple languages and corpora, highlighting its superior performance, especially in handling poorly formatted text. Finally, they discuss ethical considerations and limitations of their approach.
这篇研究论文介绍了一种名为 Segment Any Text(SAT)的新型句子分割模型,其性能超越了现有方法。SAT 通过在训练过程中减少对标点符号的依赖,实现了更强的鲁棒性;通过参数高效的微调适应不同领域(如歌词、法律文本),展现了优异的适应性;并以高效性为特点,在性能上甚至超过了强大的大型语言模型(LLMs)。作者详细描述了 SAT 的架构、训练过程以及在多种语言和语料库上的广泛评估,尤其是在处理格式较差的文本时表现出色。最后,论文讨论了该方法的伦理考量和局限性。
原文链接:https://arxiv.org/abs/2406.16678
Seventy3: 用NotebookLM将论文生成播客,让大家跟着AI一起进步。
今天的主题是:Segment Any Text: A Universal Approach for Robust, Efficient and Adaptable Sentence SegmentationSummary
This research paper introduces Segment Any Text (SAT), a novel sentence segmentation model that surpasses existing methods. SAT achieves robustness by reducing reliance on punctuation during training, demonstrates adaptability through parameter-efficient fine-tuning across diverse domains (e.g., lyrics, legal texts), and boasts high efficiency, outperforming even strong large language models (LLMs). The authors detail SAT's architecture, training process, and extensive evaluation across multiple languages and corpora, highlighting its superior performance, especially in handling poorly formatted text. Finally, they discuss ethical considerations and limitations of their approach.
这篇研究论文介绍了一种名为 Segment Any Text(SAT)的新型句子分割模型,其性能超越了现有方法。SAT 通过在训练过程中减少对标点符号的依赖,实现了更强的鲁棒性;通过参数高效的微调适应不同领域(如歌词、法律文本),展现了优异的适应性;并以高效性为特点,在性能上甚至超过了强大的大型语言模型(LLMs)。作者详细描述了 SAT 的架构、训练过程以及在多种语言和语料库上的广泛评估,尤其是在处理格式较差的文本时表现出色。最后,论文讨论了该方法的伦理考量和局限性。
原文链接:https://arxiv.org/abs/2406.16678