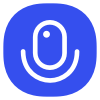
Sign up to save your podcasts
Or
Seventy3: 用NotebookLM将论文生成播客,让大家跟着AI一起进步。
今天的主题是:SPaR: Self-Play with Tree-Search Refinement to Improve Instruction-Following in Large Language ModelsSummary
This research introduces SPAR, a self-play framework using tree-search refinement to improve instruction-following in large language models (LLMs). SPAR addresses the limitations of existing methods by generating comparable preference pairs free from irrelevant variations, focusing on key differences crucial for successful instruction-following. Experiments demonstrate SPAR's effectiveness in enhancing various LLMs, surpassing GPT-4-Turbo on the IFEval benchmark in some cases. The framework iteratively improves both the LLM's responses and its ability to judge those responses. The code and data are publicly available.
本研究提出了SPAR,一种通过树搜索优化来改进大型语言模型(LLMs)指令遵循能力的自博弈框架。SPAR 通过生成不受无关变化影响的可比较偏好对,聚焦于关键差异,从而克服了现有方法的局限性,这些关键差异对于成功执行指令至关重要。实验表明,SPAR 在增强各种 LLMs 方面表现出色,在某些情况下,甚至在 IFEval 基准测试上超越了 GPT-4-Turbo。该框架能够迭代地改进模型的回答能力以及对回答的评判能力。相关代码和数据已公开提供。
原文链接:https://www.arxiv.org/abs/2412.11605
Seventy3: 用NotebookLM将论文生成播客,让大家跟着AI一起进步。
今天的主题是:SPaR: Self-Play with Tree-Search Refinement to Improve Instruction-Following in Large Language ModelsSummary
This research introduces SPAR, a self-play framework using tree-search refinement to improve instruction-following in large language models (LLMs). SPAR addresses the limitations of existing methods by generating comparable preference pairs free from irrelevant variations, focusing on key differences crucial for successful instruction-following. Experiments demonstrate SPAR's effectiveness in enhancing various LLMs, surpassing GPT-4-Turbo on the IFEval benchmark in some cases. The framework iteratively improves both the LLM's responses and its ability to judge those responses. The code and data are publicly available.
本研究提出了SPAR,一种通过树搜索优化来改进大型语言模型(LLMs)指令遵循能力的自博弈框架。SPAR 通过生成不受无关变化影响的可比较偏好对,聚焦于关键差异,从而克服了现有方法的局限性,这些关键差异对于成功执行指令至关重要。实验表明,SPAR 在增强各种 LLMs 方面表现出色,在某些情况下,甚至在 IFEval 基准测试上超越了 GPT-4-Turbo。该框架能够迭代地改进模型的回答能力以及对回答的评判能力。相关代码和数据已公开提供。
原文链接:https://www.arxiv.org/abs/2412.11605