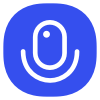
Sign up to save your podcasts
Or
Seventy3: 用NotebookLM将论文生成播客,让大家跟着AI一起进步。
今天的主题是:GReaTer: Gradients over Reasoning Makes Smaller Language Models Strong Prompt OptimizersSummary
The paper introduces GREATER, a novel prompt optimization technique for smaller language models. Unlike existing methods that rely on large, expensive LLMs for feedback, GREATER uses gradient information directly from the task loss to refine prompts. This allows smaller models to achieve performance comparable to or exceeding that of larger models on various reasoning tasks. Extensive experiments on datasets like BBH, GSM8K, and FOLIO demonstrate GREATER's superior performance and prompt transferability across different models. The approach incorporates reasoning chains for more accurate gradient calculations, significantly improving optimization compared to text-based feedback methods.
本文介绍了GREATER,一种针对小型语言模型的新型提示优化技术。与依赖大型、昂贵的语言模型(LLMs)提供反馈的现有方法不同,GREATER 直接利用任务损失的梯度信息来优化提示。这使得小型模型在多种推理任务中的表现可以媲美甚至超越大型模型。针对 BBH、GSM8K 和 FOLIO 等数据集的大量实验表明,GREATER 在性能和提示的跨模型迁移性方面表现优异。该方法结合了推理链,以实现更精确的梯度计算,相较于基于文本反馈的方法,显著提升了优化效果。
原文链接:https://arxiv.org/abs/2412.09722
Seventy3: 用NotebookLM将论文生成播客,让大家跟着AI一起进步。
今天的主题是:GReaTer: Gradients over Reasoning Makes Smaller Language Models Strong Prompt OptimizersSummary
The paper introduces GREATER, a novel prompt optimization technique for smaller language models. Unlike existing methods that rely on large, expensive LLMs for feedback, GREATER uses gradient information directly from the task loss to refine prompts. This allows smaller models to achieve performance comparable to or exceeding that of larger models on various reasoning tasks. Extensive experiments on datasets like BBH, GSM8K, and FOLIO demonstrate GREATER's superior performance and prompt transferability across different models. The approach incorporates reasoning chains for more accurate gradient calculations, significantly improving optimization compared to text-based feedback methods.
本文介绍了GREATER,一种针对小型语言模型的新型提示优化技术。与依赖大型、昂贵的语言模型(LLMs)提供反馈的现有方法不同,GREATER 直接利用任务损失的梯度信息来优化提示。这使得小型模型在多种推理任务中的表现可以媲美甚至超越大型模型。针对 BBH、GSM8K 和 FOLIO 等数据集的大量实验表明,GREATER 在性能和提示的跨模型迁移性方面表现优异。该方法结合了推理链,以实现更精确的梯度计算,相较于基于文本反馈的方法,显著提升了优化效果。
原文链接:https://arxiv.org/abs/2412.09722