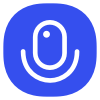
Sign up to save your podcasts
Or
Seventy3: 用NotebookML将论文生成播客,让大家跟着AI一起进步。
今天的主题是:Empirical Evaluation of Gated Recurrent Neural Networks on Sequence ModelingSource: "Empirical Evaluation of Gated Recurrent Neural Networks on Sequence Modeling" by Junyoung Chung, Caglar Gulcehre, KyungHyun Cho, and Yoshua Bengio.
Main Focus: This paper compares the performance of different recurrent neural network (RNN) units, specifically focusing on gated units: Long Short-Term Memory (LSTM) and Gated Recurrent Unit (GRU), against the traditional tanh unit.
Key Findings:
Important Ideas & Facts:
Future Directions:
The authors acknowledge the preliminary nature of their study and suggest further research to:
Overall, the paper highlights the significant advantages of gated recurrent units (LSTM & GRU) for sequence modeling tasks, showcasing their superiority over traditional RNNs in capturing long-term dependencies and achieving faster, more effective learning.
原文链接:arxiv.org
Seventy3: 用NotebookML将论文生成播客,让大家跟着AI一起进步。
今天的主题是:Empirical Evaluation of Gated Recurrent Neural Networks on Sequence ModelingSource: "Empirical Evaluation of Gated Recurrent Neural Networks on Sequence Modeling" by Junyoung Chung, Caglar Gulcehre, KyungHyun Cho, and Yoshua Bengio.
Main Focus: This paper compares the performance of different recurrent neural network (RNN) units, specifically focusing on gated units: Long Short-Term Memory (LSTM) and Gated Recurrent Unit (GRU), against the traditional tanh unit.
Key Findings:
Important Ideas & Facts:
Future Directions:
The authors acknowledge the preliminary nature of their study and suggest further research to:
Overall, the paper highlights the significant advantages of gated recurrent units (LSTM & GRU) for sequence modeling tasks, showcasing their superiority over traditional RNNs in capturing long-term dependencies and achieving faster, more effective learning.
原文链接:arxiv.org