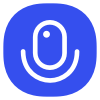
Sign up to save your podcasts
Or
Seventy3: 用NotebookML将论文生成播客,让大家跟着AI一起进步。
今天的主题是:NeRF: Representing Scenes as Neural Radiance Fields for View Synthesis - A Detailed BriefingThis briefing document reviews the key themes and findings presented in the paper "NeRF: Representing Scenes as Neural Radiance Fields for View Synthesis" by Ben Mildenhall et al.
Core Idea: The paper introduces NeRF, a novel approach for synthesizing novel views of complex scenes. NeRF utilizes a fully connected neural network to represent a scene as a continuous 5D function, mapping 3D spatial locations (x, y, z) and 2D viewing directions (θ, φ) to color (RGB) and volume density (σ).
Key Innovations:
Experimental Results: The paper presents extensive quantitative and qualitative results demonstrating NeRF’s superiority over state-of-the-art view synthesis methods on various synthetic and real-world datasets.
Key Advantages:
Limitations and Future Directions:
Conclusion: NeRF presents a significant advancement in view synthesis by introducing a novel continuous scene representation and differentiable rendering pipeline. The method's ability to generate highly detailed and photorealistic novel views from posed images holds great promise for future applications in various fields. However, addressing the limitations related to computational cost and interpretability will be crucial for wider adoption and further research.
原文链接:https://arxiv.org/abs/2003.08934
Seventy3: 用NotebookML将论文生成播客,让大家跟着AI一起进步。
今天的主题是:NeRF: Representing Scenes as Neural Radiance Fields for View Synthesis - A Detailed BriefingThis briefing document reviews the key themes and findings presented in the paper "NeRF: Representing Scenes as Neural Radiance Fields for View Synthesis" by Ben Mildenhall et al.
Core Idea: The paper introduces NeRF, a novel approach for synthesizing novel views of complex scenes. NeRF utilizes a fully connected neural network to represent a scene as a continuous 5D function, mapping 3D spatial locations (x, y, z) and 2D viewing directions (θ, φ) to color (RGB) and volume density (σ).
Key Innovations:
Experimental Results: The paper presents extensive quantitative and qualitative results demonstrating NeRF’s superiority over state-of-the-art view synthesis methods on various synthetic and real-world datasets.
Key Advantages:
Limitations and Future Directions:
Conclusion: NeRF presents a significant advancement in view synthesis by introducing a novel continuous scene representation and differentiable rendering pipeline. The method's ability to generate highly detailed and photorealistic novel views from posed images holds great promise for future applications in various fields. However, addressing the limitations related to computational cost and interpretability will be crucial for wider adoption and further research.
原文链接:https://arxiv.org/abs/2003.08934