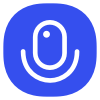
Sign up to save your podcasts
Or
This research addresses the challenge of improving monocular depth estimation (MDE) using unlabeled data through a novel distillation framework. The core innovation is Cross-Context Distillation, which combines local and global depth cues to enhance pseudo-label quality and model accuracy. A multi-teacher distillation approach further leverages complementary strengths of different depth estimation models for more robust predictions. The paper systematically analyzes the impact of various depth normalization strategies on pseudo-label distillation, revealing that Cross-Context Distillation significantly outperforms existing methods on benchmark datasets.Experiments validate the effectiveness of their approach, improving both fine details and overall depth consistency in MDE.
This research addresses the challenge of improving monocular depth estimation (MDE) using unlabeled data through a novel distillation framework. The core innovation is Cross-Context Distillation, which combines local and global depth cues to enhance pseudo-label quality and model accuracy. A multi-teacher distillation approach further leverages complementary strengths of different depth estimation models for more robust predictions. The paper systematically analyzes the impact of various depth normalization strategies on pseudo-label distillation, revealing that Cross-Context Distillation significantly outperforms existing methods on benchmark datasets.Experiments validate the effectiveness of their approach, improving both fine details and overall depth consistency in MDE.