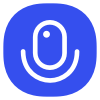
Sign up to save your podcasts
Or
This white paper from February 2025, authored by Lee Boonstra with contributions from several others, offers a detailed exploration of prompt engineering for large language models. It begins by defining prompt engineering and discussing the configuration of LLM outputs, such as length and sampling controls like temperature and top-K/top-P. The paper then examines various prompting techniques, including zero-shot, one-shot, few-shot, system, contextual, and role prompting, along with more advanced methods like step-back, Chain of Thought, self-consistency, Tree of Thoughts, and ReAct. Furthermore, it covers automatic prompt engineering and code prompting, providing practical examples for writing, explaining, translating, debugging, and reviewing code. Finally, the paper concludes with best practices for crafting effective prompts and a summary of the discussed techniques.
This white paper from February 2025, authored by Lee Boonstra with contributions from several others, offers a detailed exploration of prompt engineering for large language models. It begins by defining prompt engineering and discussing the configuration of LLM outputs, such as length and sampling controls like temperature and top-K/top-P. The paper then examines various prompting techniques, including zero-shot, one-shot, few-shot, system, contextual, and role prompting, along with more advanced methods like step-back, Chain of Thought, self-consistency, Tree of Thoughts, and ReAct. Furthermore, it covers automatic prompt engineering and code prompting, providing practical examples for writing, explaining, translating, debugging, and reviewing code. Finally, the paper concludes with best practices for crafting effective prompts and a summary of the discussed techniques.