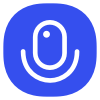
Sign up to save your podcasts
Or
This episode explores a novel method for enhancing large language models (LLMs) through "self-reflection."
Researchers have devised a technique that allows LLMs to analyze and predict their own behavior, resulting in improved accuracy and reliability. This approach, achieved by fine-tuning LLMs on datasets containing both correct and incorrect responses alongside explanations, fosters increased transparency and trust in AI systems.
By enabling LLMs to generate explanations and anticipate errors, this method contributes significantly to the development of more self-aware and reliable AI technologies.
This episode explores a novel method for enhancing large language models (LLMs) through "self-reflection."
Researchers have devised a technique that allows LLMs to analyze and predict their own behavior, resulting in improved accuracy and reliability. This approach, achieved by fine-tuning LLMs on datasets containing both correct and incorrect responses alongside explanations, fosters increased transparency and trust in AI systems.
By enabling LLMs to generate explanations and anticipate errors, this method contributes significantly to the development of more self-aware and reliable AI technologies.