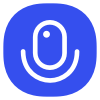
Sign up to save your podcasts
Or
The sources propose a novel approach to artificial intelligence (AI) where agents achieve strategic competence, specifically reaching Nash equilibrium, without needing task-specific post-training or fine-tuning. This is hypothesized to occur through sophisticated reasoning abilities derived from extensive pre-training combined with Bayesian learning to adapt to new situations. This research aims to advance AI autonomy and generalization, offer greater efficiency in development, and potentially bridge gaps in economic and game-theoretic modeling. Significant challenges remain, such as defining sufficient pre-training and ensuring robust convergence in complex, real-world scenarios
The sources propose a novel approach to artificial intelligence (AI) where agents achieve strategic competence, specifically reaching Nash equilibrium, without needing task-specific post-training or fine-tuning. This is hypothesized to occur through sophisticated reasoning abilities derived from extensive pre-training combined with Bayesian learning to adapt to new situations. This research aims to advance AI autonomy and generalization, offer greater efficiency in development, and potentially bridge gaps in economic and game-theoretic modeling. Significant challenges remain, such as defining sufficient pre-training and ensuring robust convergence in complex, real-world scenarios