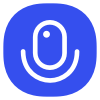
Sign up to save your podcasts
Or
Today on the podcast, we have data expert Oliver Graeser who is the Head of Data & Insights at Parcel Perform.
Our goal today is to give everyone a 101 on Data and to dissect the difference between Data Modeling/Analytics, Data Lakes and so on.
Questions for Oliver - background
1. Could you please talk about your background? What got you interested in technology and in particular data analytics?
2. You transitioned from the banking/quant world into a pure tech role. What inspired this change?
Data 101
3. Can we start off with a basic 101 on Data? Could you please break down all of this for us or put it into context? What is the relationship between Data Science/Data Analytics/Data Modeling/Artificial Intelligence and Machine Learning?
4. Could you paint a rough flowchart on how data progresses from one stage to another? We hear of terms like Data Lake, Data Buckets and so on. For the average person who does not know much about how data is collected and processed, could you give us an overview of this?
5. We know what a relational database is (like SQL Database). Why would one need a non-relational database? Also, what is No SQL?
6. We also hear about technologies like Hadoop, MongoDB, etc. What are the applications of these kinds of technologies?
Data Science and Predictive Analytics
7. Could you give a real-world example of the application of Data Science?
8. What does Parcel Perform do? How do these concepts relating to data apply to Parcel Perform's workflow?
9. What is the difference between good predictive analytics and AI?
10. On the subject of AI, what is LLM and where are the situations in which one would need them? How does data science tie into LLMs?
Future of AI/Data
11. In your opinion, how much of what we hear about AI is a fad vs something to be seriously considered and concerned about?
12. How is AI shaping your line of work?
13. What can engineers do today to not be replaced by AI in the future?
Advice for budding technologists
14. What is your advice to budding technologists graduating from universities and wishing to pursue something in data science?
Today on the podcast, we have data expert Oliver Graeser who is the Head of Data & Insights at Parcel Perform.
Our goal today is to give everyone a 101 on Data and to dissect the difference between Data Modeling/Analytics, Data Lakes and so on.
Questions for Oliver - background
1. Could you please talk about your background? What got you interested in technology and in particular data analytics?
2. You transitioned from the banking/quant world into a pure tech role. What inspired this change?
Data 101
3. Can we start off with a basic 101 on Data? Could you please break down all of this for us or put it into context? What is the relationship between Data Science/Data Analytics/Data Modeling/Artificial Intelligence and Machine Learning?
4. Could you paint a rough flowchart on how data progresses from one stage to another? We hear of terms like Data Lake, Data Buckets and so on. For the average person who does not know much about how data is collected and processed, could you give us an overview of this?
5. We know what a relational database is (like SQL Database). Why would one need a non-relational database? Also, what is No SQL?
6. We also hear about technologies like Hadoop, MongoDB, etc. What are the applications of these kinds of technologies?
Data Science and Predictive Analytics
7. Could you give a real-world example of the application of Data Science?
8. What does Parcel Perform do? How do these concepts relating to data apply to Parcel Perform's workflow?
9. What is the difference between good predictive analytics and AI?
10. On the subject of AI, what is LLM and where are the situations in which one would need them? How does data science tie into LLMs?
Future of AI/Data
11. In your opinion, how much of what we hear about AI is a fad vs something to be seriously considered and concerned about?
12. How is AI shaping your line of work?
13. What can engineers do today to not be replaced by AI in the future?
Advice for budding technologists
14. What is your advice to budding technologists graduating from universities and wishing to pursue something in data science?