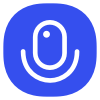
Sign up to save your podcasts
Or
This week we’re wondering how an algorithm might be able to explain itself. And we’re joined by David Watson. David is a Doctoral candidate at the Oxford Internet Institute. He focusses on the epistemological foundations of machine learning and used to be a data scientist at Queen Mary’s Centre for Translational Bioinformatics.
Before we’ve spoken about the ethics of different automated systems making decisions, whether that’s decisions that relate to policing, healthcare, justice or finance. How can we understand that decision? How can we ensure that the decision was fair and unbiased? There is both a legal and a technical aspect of explainability. The legal aspect asks how we audit systems and uphold the algorithms that organisations build. The technical aspect asks how we build explainability into our systems.
David mentioned some papers about medical applications. He suggests the following papers to take a look at [1] and [2]
We talked about FATML – the organisation that looks into fairness, accountability and transparency in Machine Learning. Here is their website.
Books we like: Cathy O’Neil with Weapons of Math Destruction, Safiya Noble with Algorithms of Oppression and Virginia Eubanks with
This week we’re wondering how an algorithm might be able to explain itself. And we’re joined by David Watson. David is a Doctoral candidate at the Oxford Internet Institute. He focusses on the epistemological foundations of machine learning and used to be a data scientist at Queen Mary’s Centre for Translational Bioinformatics.
Before we’ve spoken about the ethics of different automated systems making decisions, whether that’s decisions that relate to policing, healthcare, justice or finance. How can we understand that decision? How can we ensure that the decision was fair and unbiased? There is both a legal and a technical aspect of explainability. The legal aspect asks how we audit systems and uphold the algorithms that organisations build. The technical aspect asks how we build explainability into our systems.
David mentioned some papers about medical applications. He suggests the following papers to take a look at [1] and [2]
We talked about FATML – the organisation that looks into fairness, accountability and transparency in Machine Learning. Here is their website.
Books we like: Cathy O’Neil with Weapons of Math Destruction, Safiya Noble with Algorithms of Oppression and Virginia Eubanks with