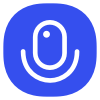
Sign up to save your podcasts
Or
We talked about the scaling of startups.
Recently, Paul Graham tweeted that scaling the startup prematurely is the single most dangerous mistake founders can make. He also mentioned two causes: wishful thinking on the part of founders & pushing to scale on the part of investors.
However, there's no definition of what it means to "scale prematurely". We propose an approximate definition: "to increase the bet size without a proof of positive expectation".
This definition implies that the founders need to produce a "proof" that their startup has a positive expectation of profit. How can they do it?
Broadly speaking, there are two ways:
* Extrapolate from data
* Extract axioms from data, then produce a proof using the axioms
There is a fundamental difference between two methods:
* The first method takes an "average" of all available data.
* The second method filters the dataset to include only the data that has "never failed" (is not random)
Additionally, the second method uses the external data - all other axioms from all branches of science.
We think the second method is superior, but it takes more time. Additionally, it may be necessary to introduce additional axioms to complete the proof. Such additional axioms may have low "support" (i.e. occur in data only a few times), so they can be invalidated in the near future.
However, with the recent advances in machine learning, it's conceivable to have an AI agent that could prove the theorems quickly. This would open the way to using the second approach.
Looking forward!
We talked about the scaling of startups.
Recently, Paul Graham tweeted that scaling the startup prematurely is the single most dangerous mistake founders can make. He also mentioned two causes: wishful thinking on the part of founders & pushing to scale on the part of investors.
However, there's no definition of what it means to "scale prematurely". We propose an approximate definition: "to increase the bet size without a proof of positive expectation".
This definition implies that the founders need to produce a "proof" that their startup has a positive expectation of profit. How can they do it?
Broadly speaking, there are two ways:
* Extrapolate from data
* Extract axioms from data, then produce a proof using the axioms
There is a fundamental difference between two methods:
* The first method takes an "average" of all available data.
* The second method filters the dataset to include only the data that has "never failed" (is not random)
Additionally, the second method uses the external data - all other axioms from all branches of science.
We think the second method is superior, but it takes more time. Additionally, it may be necessary to introduce additional axioms to complete the proof. Such additional axioms may have low "support" (i.e. occur in data only a few times), so they can be invalidated in the near future.
However, with the recent advances in machine learning, it's conceivable to have an AI agent that could prove the theorems quickly. This would open the way to using the second approach.
Looking forward!